- Data Science
- Data Analysis
- Data Visualization
- Machine Learning
- Deep Learning
- Computer Vision
- Artificial Intelligence
- AI ML DS Interview Series
- AI ML DS Projects series
- Data Engineering
- Web Scrapping

Data Analysis in Research: Types & Methods
Data analysis is a crucial step in the research process, transforming raw data into meaningful insights that drive informed decisions and advance knowledge. This article explores the various types and methods of data analysis in research, providing a comprehensive guide for researchers across disciplines.
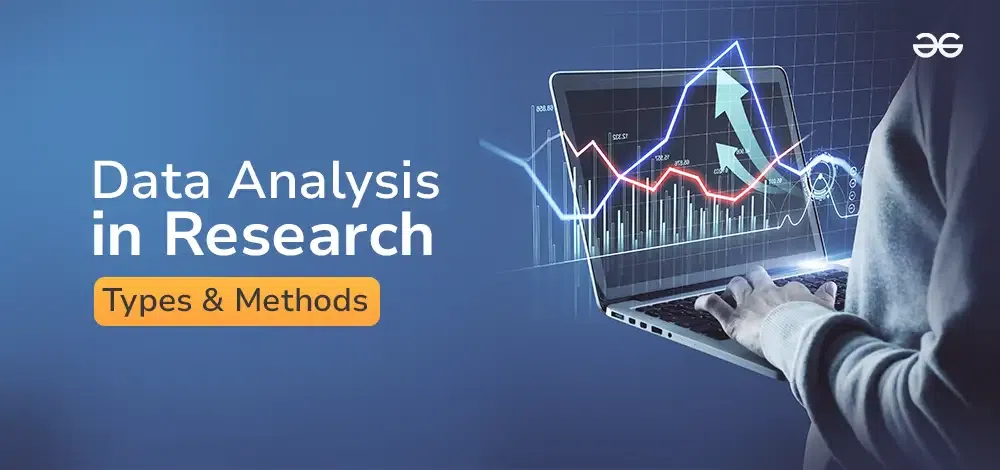
Overview of Data analysis in research
Data analysis in research is the systematic use of statistical and analytical tools to describe, summarize, and draw conclusions from datasets. This process involves organizing, analyzing, modeling, and transforming data to identify trends, establish connections, and inform decision-making. The main goals include describing data through visualization and statistics, making inferences about a broader population, predicting future events using historical data, and providing data-driven recommendations. The stages of data analysis involve collecting relevant data, preprocessing to clean and format it, conducting exploratory data analysis to identify patterns, building and testing models, interpreting results, and effectively reporting findings.
- Main Goals : Describe data, make inferences, predict future events, and provide data-driven recommendations.
- Stages of Data Analysis : Data collection, preprocessing, exploratory data analysis, model building and testing, interpretation, and reporting.
Types of Data Analysis
1. descriptive analysis.
Descriptive analysis focuses on summarizing and describing the features of a dataset. It provides a snapshot of the data, highlighting central tendencies, dispersion, and overall patterns.
- Central Tendency Measures : Mean, median, and mode are used to identify the central point of the dataset.
- Dispersion Measures : Range, variance, and standard deviation help in understanding the spread of the data.
- Frequency Distribution : This shows how often each value in a dataset occurs.
2. Inferential Analysis
Inferential analysis allows researchers to make predictions or inferences about a population based on a sample of data. It is used to test hypotheses and determine the relationships between variables.
- Hypothesis Testing : Techniques like t-tests, chi-square tests, and ANOVA are used to test assumptions about a population.
- Regression Analysis : This method examines the relationship between dependent and independent variables.
- Confidence Intervals : These provide a range of values within which the true population parameter is expected to lie.
3. Exploratory Data Analysis (EDA)
EDA is an approach to analyzing data sets to summarize their main characteristics, often with visual methods. It helps in discovering patterns, spotting anomalies, and checking assumptions with the help of graphical representations.
- Visual Techniques : Histograms, box plots, scatter plots, and bar charts are commonly used in EDA.
- Summary Statistics : Basic statistical measures are used to describe the dataset.
4. Predictive Analysis
Predictive analysis uses statistical techniques and machine learning algorithms to predict future outcomes based on historical data.
- Machine Learning Models : Algorithms like linear regression, decision trees, and neural networks are employed to make predictions.
- Time Series Analysis : This method analyzes data points collected or recorded at specific time intervals to forecast future trends.
5. Causal Analysis
Causal analysis aims to identify cause-and-effect relationships between variables. It helps in understanding the impact of one variable on another.
- Experiments : Controlled experiments are designed to test the causality.
- Quasi-Experimental Designs : These are used when controlled experiments are not feasible.
6. Mechanistic Analysis
Mechanistic analysis seeks to understand the underlying mechanisms or processes that drive observed phenomena. It is common in fields like biology and engineering.
Methods of Data Analysis
1. quantitative methods.
Quantitative methods involve numerical data and statistical analysis to uncover patterns, relationships, and trends.
- Statistical Analysis : Includes various statistical tests and measures.
- Mathematical Modeling : Uses mathematical equations to represent relationships among variables.
- Simulation : Computer-based models simulate real-world processes to predict outcomes.
2. Qualitative Methods
Qualitative methods focus on non-numerical data, such as text, images, and audio, to understand concepts, opinions, or experiences.
- Content Analysis : Systematic coding and categorizing of textual information.
- Thematic Analysis : Identifying themes and patterns within qualitative data.
- Narrative Analysis : Examining the stories or accounts shared by participants.
3. Mixed Methods
Mixed methods combine both quantitative and qualitative approaches to provide a more comprehensive analysis.
- Sequential Explanatory Design : Quantitative data is collected and analyzed first, followed by qualitative data to explain the quantitative results.
- Concurrent Triangulation Design : Both qualitative and quantitative data are collected simultaneously but analyzed separately to compare results.
4. Data Mining
Data mining involves exploring large datasets to discover patterns and relationships.
- Clustering : Grouping data points with similar characteristics.
- Association Rule Learning : Identifying interesting relations between variables in large databases.
- Classification : Assigning items to predefined categories based on their attributes.
5. Big Data Analytics
Big data analytics involves analyzing vast amounts of data to uncover hidden patterns, correlations, and other insights.
- Hadoop and Spark : Frameworks for processing and analyzing large datasets.
- NoSQL Databases : Designed to handle unstructured data.
- Machine Learning Algorithms : Used to analyze and predict complex patterns in big data.
Applications and Case Studies
Numerous fields and industries use data analysis methods, which provide insightful information and facilitate data-driven decision-making. The following case studies demonstrate the effectiveness of data analysis in research:
Medical Care:
- Predicting Patient Readmissions: By using data analysis to create predictive models, healthcare facilities may better identify patients who are at high risk of readmission and implement focused interventions to enhance patient care.
- Disease Outbreak Analysis: Researchers can monitor and forecast disease outbreaks by examining both historical and current data. This information aids public health authorities in putting preventative and control measures in place.
- Fraud Detection: To safeguard clients and lessen financial losses, financial institutions use data analysis tools to identify fraudulent transactions and activities.
- investing Strategies: By using data analysis, quantitative investing models that detect trends in stock prices may be created, assisting investors in optimizing their portfolios and making well-informed choices.
- Customer Segmentation: Businesses may divide up their client base into discrete groups using data analysis, which makes it possible to launch focused marketing efforts and provide individualized services.
- Social Media Analytics: By tracking brand sentiment, identifying influencers, and understanding consumer preferences, marketers may develop more successful marketing strategies by analyzing social media data.
- Predicting Student Performance: By using data analysis tools, educators may identify at-risk children and forecast their performance. This allows them to give individualized learning plans and timely interventions.
- Education Policy Analysis: Data may be used by researchers to assess the efficacy of policies, initiatives, and programs in education, offering insights for evidence-based decision-making.
Social Science Fields:
- Opinion mining in politics: By examining public opinion data from news stories and social media platforms, academics and policymakers may get insight into prevailing political opinions and better understand how the public feels about certain topics or candidates.
- Crime Analysis: Researchers may spot trends, anticipate high-risk locations, and help law enforcement use resources wisely in order to deter and lessen crime by studying crime data.
Data analysis is a crucial step in the research process because it enables companies and researchers to glean insightful information from data. By using diverse analytical methodologies and approaches, scholars may reveal latent patterns, arrive at well-informed conclusions, and tackle intricate research inquiries. Numerous statistical, machine learning, and visualization approaches are among the many data analysis tools available, offering a comprehensive toolbox for addressing a broad variety of research problems.
Data Analysis in Research FAQs:
What are the main phases in the process of analyzing data.
In general, the steps involved in data analysis include gathering data, preparing it, doing exploratory data analysis, constructing and testing models, interpreting the results, and reporting the results. Every stage is essential to guaranteeing the analysis's efficacy and correctness.
What are the differences between the examination of qualitative and quantitative data?
In order to comprehend and analyze non-numerical data, such text, pictures, or observations, qualitative data analysis often employs content analysis, grounded theory, or ethnography. Comparatively, quantitative data analysis works with numerical data and makes use of statistical methods to identify, deduce, and forecast trends in the data.
What are a few popular statistical methods for analyzing data?
In data analysis, predictive modeling, inferential statistics, and descriptive statistics are often used. While inferential statistics establish assumptions and draw inferences about a wider population, descriptive statistics highlight the fundamental characteristics of the data. To predict unknown values or future events, predictive modeling is used.
In what ways might data analysis methods be used in the healthcare industry?
In the healthcare industry, data analysis may be used to optimize treatment regimens, monitor disease outbreaks, forecast patient readmissions, and enhance patient care. It is also essential for medication development, clinical research, and the creation of healthcare policies.
What difficulties may one encounter while analyzing data?
Answer: Typical problems with data quality include missing values, outliers, and biased samples, all of which may affect how accurate the analysis is. Furthermore, it might be computationally demanding to analyze big and complicated datasets, necessitating certain tools and knowledge. It's also critical to handle ethical issues, such as data security and privacy.
Similar Reads
- Data Analysis in Research: Types & Methods Data analysis is a crucial step in the research process, transforming raw data into meaningful insights that drive informed decisions and advance knowledge. This article explores the various types and methods of data analysis in research, providing a comprehensive guide for researchers across discip 7 min read
- Types of Data Analysis Techniques Data analysis techniques have significantly evolved, providing a comprehensive toolkit for understanding, interpreting, and predicting data patterns. These methods are crucial in extracting actionable insights from data, enabling organizations to make informed decisions. This article will cover majo 7 min read
- Types of Research Methods Explained with Examples Research methods are the various strategies, techniques, and tools that researchers use to collect and analyze data. These methods help researchers find answers to their questions and gain a better understanding of different topics. Whether conducting experiments, surveys, or interviews, choosing th 7 min read
- Types of Statistical Data Analysis Statistics data analysis is a class of analysis that includes different techniques and methods for collection, data analysis, interpretation and presentation of data. Knowing the approach to data analysis is one of the crucial aspects that allows drawing a meaningful conclusion. In this article, the 7 min read
- GRE Data Analysis | Methods for Presenting Data Data Interpretation Question in GRE will require the knowledge of understanding Data Representation methods. It is very Important to understand data correctly and apply the same to solve the problem. Important Terms to know - Variable: A characteristics which varies with respect to given categories. 3 min read
- What is Data Analysis? Data analysis is an essential aspect of modern decision-making processes across various sectors, including business, healthcare, finance, and academia. As organizations generate massive amounts of data daily, understanding how to extract meaningful insights from this data becomes crucial. In this ar 13 min read
- How to Write Data Analysis Reports Reports on data analysis are essential for communicating data-driven insights to decision-makers, stakeholders, and other pertinent parties. These reports provide an organized format for providing conclusions, analyses, and suggestions derived from data set analysis. In this guide, we will learn how 9 min read
- Types of Business Research What is Business Research?Business Research represents a structured approach to collecting appropriate information concerning a firm's operations to optimize profits and facilitate well-informed decision-making. This process includes acquiring comprehensive data about diverse aspects of business, in 13 min read
- Methods of Marketing Research Marketing Research is defined as a systematic and comprehensive process of gathering, analysing, and interpreting information to gain insights into various aspects of marketing, such as market trends, consumer behaviour, competition, and product or service performance. It plays a pivotal role in gui 12 min read
- Data Analysis Examples Data analysis stands as the cornerstone of informed decision-making in today's data-driven world, driving innovation and yielding actionable insights across industries. From healthcare and finance to retail and urban planning, the applications of data analysis are vast and transformative. In this in 7 min read
- Exploratory Data Analysis (EDA) - Types and Tools Exploratory data analysis was promoted by John Tukey to encourage statisticians to explore data, and possibly formulate hypotheses that might cause new data collection and experiments. EDA focuses more narrowly on checking assumptions required for model fitting and hypothesis testing. It also checks 6 min read
- Different Types of Data Sampling Methods and Techniques Data sampling is a statistical method that involves selecting a part of a population of data to create representative samples. The fundamental aim is to draw conclusions about the entire population without having to engage with every individual data point, thus saving time, resources, and effort whi 14 min read
- Why Data Analysis is Important? DData Analysis involves inspecting, transforming, and modeling data to discover useful information, inform conclusions, and support decision-making. It encompasses a range of techniques and tools used to interpret raw data, identify patterns, and extract actionable insights. Effective data analysis 5 min read
- Types of Legal Research Legal research is like detectives' work for lawyers. They search for information about laws, court cases, and legal rules to find answers to their questions. This helps them understand the law better, solve legal problems, and make strong arguments in court. Lawyers need to do this research so they 9 min read
- Top 10 SQL Projects For Data Analysis SQL stands for Structured Query Language and is a standard database programming language that is used in data analysis and to access data in databases. It is a popular query language that is used in all types of devices. SQL is a fundamental tool for data scientists to extract, manipulate, and analy 9 min read
- What is Statistical Analysis in Data Science? Statistical analysis serves as a cornerstone in the field of data science, providing essential tools and techniques for understanding, interpreting, and making decisions based on data. In this article we are going to learn about the statistical analysis in data science and discuss few types of stati 6 min read
- Qualitative Data Analysis Methodologies and Methods Qualitative data analysis involves interpreting non-numerical data to identify patterns, themes, and insights. There are several methodologies and methods used in qualitative data analysis. In this article, we will explore qualitative data analysis techniques in great detail, with each method provid 15+ min read
- Steps for Mastering Exploratory Data Analysis | EDA Steps Mastering exploratory data analysis (EDA) is crucial for understanding your data, identifying patterns, and generating insights that can inform further analysis or decision-making. Data is the lifeblood of cutting-edge groups, and the capability to extract insights from records has become a crucial 15+ min read
- 10 Data Analytics Project Ideas With Data replacing everything, the art of analyzing, interpreting, and deriving use from the presented data has become a necessity in all spheres of business. The Exploration of Data Analytics Project Ideas helps as a practical avenue for applying analytical concepts, driving personal growth and or 13 min read
- AI-ML-DS Blogs
- Data Science Blogathon 2024
Improve your Coding Skills with Practice
What kind of Experience do you want to share?
- Skip to main content
- Skip to primary sidebar
- Skip to footer
- QuestionPro

- Solutions Industries Gaming Automotive Sports and events Education Government Travel & Hospitality Financial Services Healthcare Cannabis Technology Use Case AskWhy Communities Audience Contactless surveys Mobile LivePolls Member Experience GDPR Positive People Science 360 Feedback Surveys
- Resources Blog eBooks Survey Templates Case Studies Training Help center

Home Market Research
Data Analysis in Research: Types & Methods
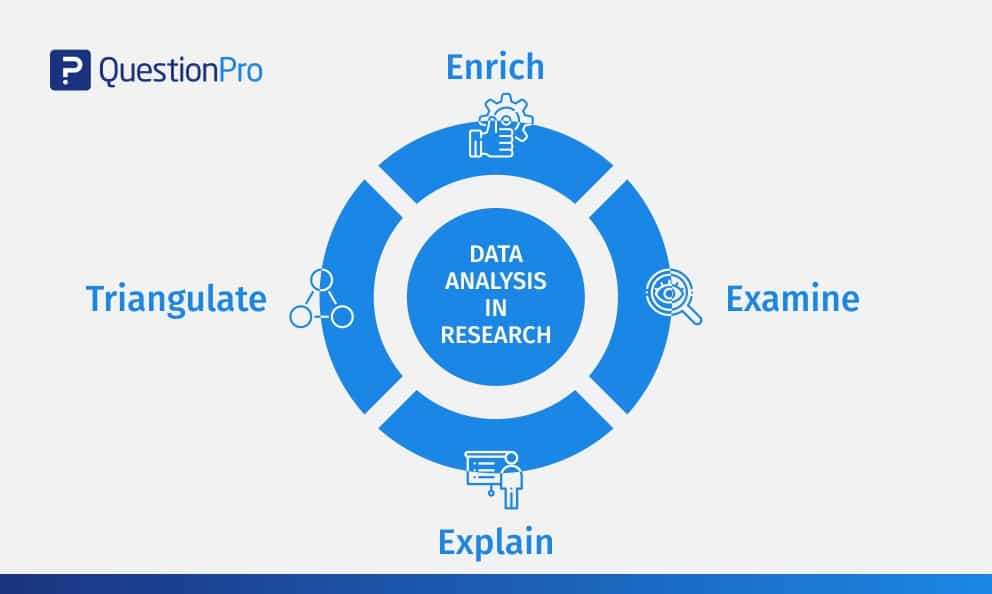
What is data analysis in research?
Definition of research in data analysis: According to LeCompte and Schensul, research data analysis is a process used by researchers to reduce data to a story and interpret it to derive insights. The data analysis process helps reduce a large chunk of data into smaller fragments, which makes sense.
Three essential things occur during the data analysis process — the first is data organization . Summarization and categorization together contribute to becoming the second known method used for data reduction. It helps find patterns and themes in the data for easy identification and linking. The third and last way is data analysis – researchers do it in both top-down and bottom-up fashion.
On the other hand, Marshall and Rossman describe data analysis as a messy, ambiguous, and time-consuming but creative and fascinating process through which a mass of collected data is brought to order, structure and meaning.
We can say that “the data analysis and data interpretation is a process representing the application of deductive and inductive logic to the research and data analysis.”
Why analyze data in research?
Researchers rely heavily on data as they have a story to tell or research problems to solve. It starts with a question, and data is nothing but an answer to that question. But, what if there is no question to ask? Well! It is possible to explore data even without a problem – we call it ‘Data Mining’, which often reveals some interesting patterns within the data that are worth exploring.
Irrelevant to the type of data researchers explore, their mission and audiences’ vision guide them to find the patterns to shape the story they want to tell. One of the essential things expected from researchers while analyzing data is to stay open and remain unbiased toward unexpected patterns, expressions, and results. Remember, sometimes, data analysis tells the most unforeseen yet exciting stories that were not expected when initiating data analysis. Therefore, rely on the data you have at hand and enjoy the journey of exploratory research.
Create a Free Account
Types of data in research
Every kind of data has a rare quality of describing things after assigning a specific value to it. For analysis, you need to organize these values, processed and presented in a given context, to make it useful. Data can be in different forms; here are the primary data types.
- Qualitative data: When the data presented has words and descriptions, then we call it qualitative data . Although you can observe this data, it is subjective and harder to analyze data in research, especially for comparison. Example: Quality data represents everything describing taste, experience, texture, or an opinion that is considered quality data. This type of data is usually collected through focus groups, personal qualitative interviews , qualitative observation or using open-ended questions in surveys.
- Quantitative data: Any data expressed in numbers of numerical figures are called quantitative data . This type of data can be distinguished into categories, grouped, measured, calculated, or ranked. Example: questions such as age, rank, cost, length, weight, scores, etc. everything comes under this type of data. You can present such data in graphical format, charts, or apply statistical analysis methods to this data. The (Outcomes Measurement Systems) OMS questionnaires in surveys are a significant source of collecting numeric data.
- Categorical data : It is data presented in groups. However, an item included in the categorical data cannot belong to more than one group. Example: A person responding to a survey by telling his living style, marital status, smoking habit, or drinking habit comes under the categorical data. A chi-square test is a standard method used to analyze this data.
Learn More : Examples of Qualitative Data in Education
Data analysis in qualitative research
Data analysis and qualitative data research work a little differently from the numerical data as the quality data is made up of words, descriptions, images, objects, and sometimes symbols. Getting insight from such complicated information is a complicated process. Hence it is typically used for exploratory research and data analysis .
Finding patterns in the qualitative data
Although there are several ways to find patterns in the textual information, a word-based method is the most relied and widely used global technique for research and data analysis. Notably, the data analysis process in qualitative research is manual. Here the researchers usually read the available data and find repetitive or commonly used words.
For example, while studying data collected from African countries to understand the most pressing issues people face, researchers might find “food” and “hunger” are the most commonly used words and will highlight them for further analysis.
The keyword context is another widely used word-based technique. In this method, the researcher tries to understand the concept by analyzing the context in which the participants use a particular keyword.
For example , researchers conducting research and data analysis for studying the concept of ‘diabetes’ amongst respondents might analyze the context of when and how the respondent has used or referred to the word ‘diabetes.’
The scrutiny-based technique is also one of the highly recommended text analysis methods used to identify a quality data pattern. Compare and contrast is the widely used method under this technique to differentiate how a specific text is similar or different from each other.
For example: To find out the “importance of resident doctor in a company,” the collected data is divided into people who think it is necessary to hire a resident doctor and those who think it is unnecessary. Compare and contrast is the best method that can be used to analyze the polls having single-answer questions types .
Metaphors can be used to reduce the data pile and find patterns in it so that it becomes easier to connect data with theory.
Variable Partitioning is another technique used to split variables so that researchers can find more coherent descriptions and explanations from the enormous data.
Methods used for data analysis in qualitative research
There are several techniques to analyze the data in qualitative research, but here are some commonly used methods,
- Content Analysis: It is widely accepted and the most frequently employed technique for data analysis in research methodology. It can be used to analyze the documented information from text, images, and sometimes from the physical items. It depends on the research questions to predict when and where to use this method.
- Narrative Analysis: This method is used to analyze content gathered from various sources such as personal interviews, field observation, and surveys . The majority of times, stories, or opinions shared by people are focused on finding answers to the research questions.
- Discourse Analysis: Similar to narrative analysis, discourse analysis is used to analyze the interactions with people. Nevertheless, this particular method considers the social context under which or within which the communication between the researcher and respondent takes place. In addition to that, discourse analysis also focuses on the lifestyle and day-to-day environment while deriving any conclusion.
- Grounded Theory: When you want to explain why a particular phenomenon happened, then using grounded theory for analyzing quality data is the best resort. Grounded theory is applied to study data about the host of similar cases occurring in different settings. When researchers are using this method, they might alter explanations or produce new ones until they arrive at some conclusion.
Choosing the right software can be tough. Whether you’re a researcher, business leader, or marketer, check out the top 10 qualitative data analysis software for analyzing qualitative data.
Data analysis in quantitative research
Preparing data for analysis.
The first stage in research and data analysis is to make it for the analysis so that the nominal data can be converted into something meaningful. Data preparation consists of the below phases.
Phase I: Data Validation
Data validation is done to understand if the collected data sample is per the pre-set standards, or it is a biased data sample again divided into four different stages
- Fraud: To ensure an actual human being records each response to the survey or the questionnaire
- Screening: To make sure each participant or respondent is selected or chosen in compliance with the research criteria
- Procedure: To ensure ethical standards were maintained while collecting the data sample
- Completeness: To ensure that the respondent has answered all the questions in an online survey. Else, the interviewer had asked all the questions devised in the questionnaire.
Phase II: Data Editing
More often, an extensive research data sample comes loaded with errors. Respondents sometimes fill in some fields incorrectly or sometimes skip them accidentally. Data editing is a process wherein the researchers have to confirm that the provided data is free of such errors. They need to conduct necessary checks and outlier checks to edit the raw edit and make it ready for analysis.
Phase III: Data Coding
Out of all three, this is the most critical phase of data preparation associated with grouping and assigning values to the survey responses . If a survey is completed with a 1000 sample size, the researcher will create an age bracket to distinguish the respondents based on their age. Thus, it becomes easier to analyze small data buckets rather than deal with the massive data pile.
LEARN ABOUT: Steps in Qualitative Research
Methods used for data analysis in quantitative research
After the data is prepared for analysis, researchers are open to using different research and data analysis methods to derive meaningful insights. For sure, statistical analysis plans are the most favored to analyze numerical data. In statistical analysis, distinguishing between categorical data and numerical data is essential, as categorical data involves distinct categories or labels, while numerical data consists of measurable quantities. The method is again classified into two groups. First, ‘Descriptive Statistics’ used to describe data. Second, ‘Inferential statistics’ that helps in comparing the data .
Descriptive statistics
This method is used to describe the basic features of versatile types of data in research. It presents the data in such a meaningful way that pattern in the data starts making sense. Nevertheless, the descriptive analysis does not go beyond making conclusions. The conclusions are again based on the hypothesis researchers have formulated so far. Here are a few major types of descriptive analysis methods.
Measures of Frequency
- Count, Percent, Frequency
- It is used to denote home often a particular event occurs.
- Researchers use it when they want to showcase how often a response is given.
Measures of Central Tendency
- Mean, Median, Mode
- The method is widely used to demonstrate distribution by various points.
- Researchers use this method when they want to showcase the most commonly or averagely indicated response.
Measures of Dispersion or Variation
- Range, Variance, Standard deviation
- Here the field equals high/low points.
- Variance standard deviation = difference between the observed score and mean
- It is used to identify the spread of scores by stating intervals.
- Researchers use this method to showcase data spread out. It helps them identify the depth until which the data is spread out that it directly affects the mean.
Measures of Position
- Percentile ranks, Quartile ranks
- It relies on standardized scores helping researchers to identify the relationship between different scores.
- It is often used when researchers want to compare scores with the average count.
For quantitative research use of descriptive analysis often give absolute numbers, but the in-depth analysis is never sufficient to demonstrate the rationale behind those numbers. Nevertheless, it is necessary to think of the best method for research and data analysis suiting your survey questionnaire and what story researchers want to tell. For example, the mean is the best way to demonstrate the students’ average scores in schools. It is better to rely on the descriptive statistics when the researchers intend to keep the research or outcome limited to the provided sample without generalizing it. For example, when you want to compare average voting done in two different cities, differential statistics are enough.
Descriptive analysis is also called a ‘univariate analysis’ since it is commonly used to analyze a single variable.
Inferential statistics
Inferential statistics are used to make predictions about a larger population after research and data analysis of the representing population’s collected sample. For example, you can ask some odd 100 audiences at a movie theater if they like the movie they are watching. Researchers then use inferential statistics on the collected sample to reason that about 80-90% of people like the movie.
Here are two significant areas of inferential statistics.
- Estimating parameters: It takes statistics from the sample research data and demonstrates something about the population parameter.
- Hypothesis test: I t’s about sampling research data to answer the survey research questions. For example, researchers might be interested to understand if the new shade of lipstick recently launched is good or not, or if the multivitamin capsules help children to perform better at games.
These are sophisticated analysis methods used to showcase the relationship between different variables instead of describing a single variable. It is often used when researchers want something beyond absolute numbers to understand the relationship between variables.
Here are some of the commonly used methods for data analysis in research.
- Correlation: When researchers are not conducting experimental research or quasi-experimental research wherein the researchers are interested to understand the relationship between two or more variables, they opt for correlational research methods.
- Cross-tabulation: Also called contingency tables, cross-tabulation is used to analyze the relationship between multiple variables. Suppose provided data has age and gender categories presented in rows and columns. A two-dimensional cross-tabulation helps for seamless data analysis and research by showing the number of males and females in each age category.
- Regression analysis: For understanding the strong relationship between two variables, researchers do not look beyond the primary and commonly used regression analysis method, which is also a type of predictive analysis used. In this method, you have an essential factor called the dependent variable. You also have multiple independent variables in regression analysis. You undertake efforts to find out the impact of independent variables on the dependent variable. The values of both independent and dependent variables are assumed as being ascertained in an error-free random manner.
- Frequency tables: The statistical procedure is used for testing the degree to which two or more vary or differ in an experiment. A considerable degree of variation means research findings were significant. In many contexts, ANOVA testing and variance analysis are similar.
- Analysis of variance: The statistical procedure is used for testing the degree to which two or more vary or differ in an experiment. A considerable degree of variation means research findings were significant. In many contexts, ANOVA testing and variance analysis are similar.
Considerations in research data analysis
- Researchers must have the necessary research skills to analyze and manipulation the data , Getting trained to demonstrate a high standard of research practice. Ideally, researchers must possess more than a basic understanding of the rationale of selecting one statistical method over the other to obtain better data insights.
- Usually, research and data analytics projects differ by scientific discipline; therefore, getting statistical advice at the beginning of analysis helps design a survey questionnaire, select data collection methods , and choose samples.
LEARN ABOUT: Best Data Collection Tools
- The primary aim of data research and analysis is to derive ultimate insights that are unbiased. Any mistake in or keeping a biased mind to collect data, selecting an analysis method, or choosing audience sample il to draw a biased inference.
- Irrelevant to the sophistication used in research data and analysis is enough to rectify the poorly defined objective outcome measurements. It does not matter if the design is at fault or intentions are not clear, but lack of clarity might mislead readers, so avoid the practice.
- The motive behind data analysis in research is to present accurate and reliable data. As far as possible, avoid statistical errors, and find a way to deal with everyday challenges like outliers, missing data, data altering, data mining , or developing graphical representation.
LEARN MORE: Descriptive Research vs Correlational Research The sheer amount of data generated daily is frightening. Especially when data analysis has taken center stage. in 2018. In last year, the total data supply amounted to 2.8 trillion gigabytes. Hence, it is clear that the enterprises willing to survive in the hypercompetitive world must possess an excellent capability to analyze complex research data, derive actionable insights, and adapt to the new market needs.
LEARN ABOUT: Average Order Value
QuestionPro is an online survey platform that empowers organizations in data analysis and research and provides them a medium to collect data by creating appealing surveys.
MORE LIKE THIS
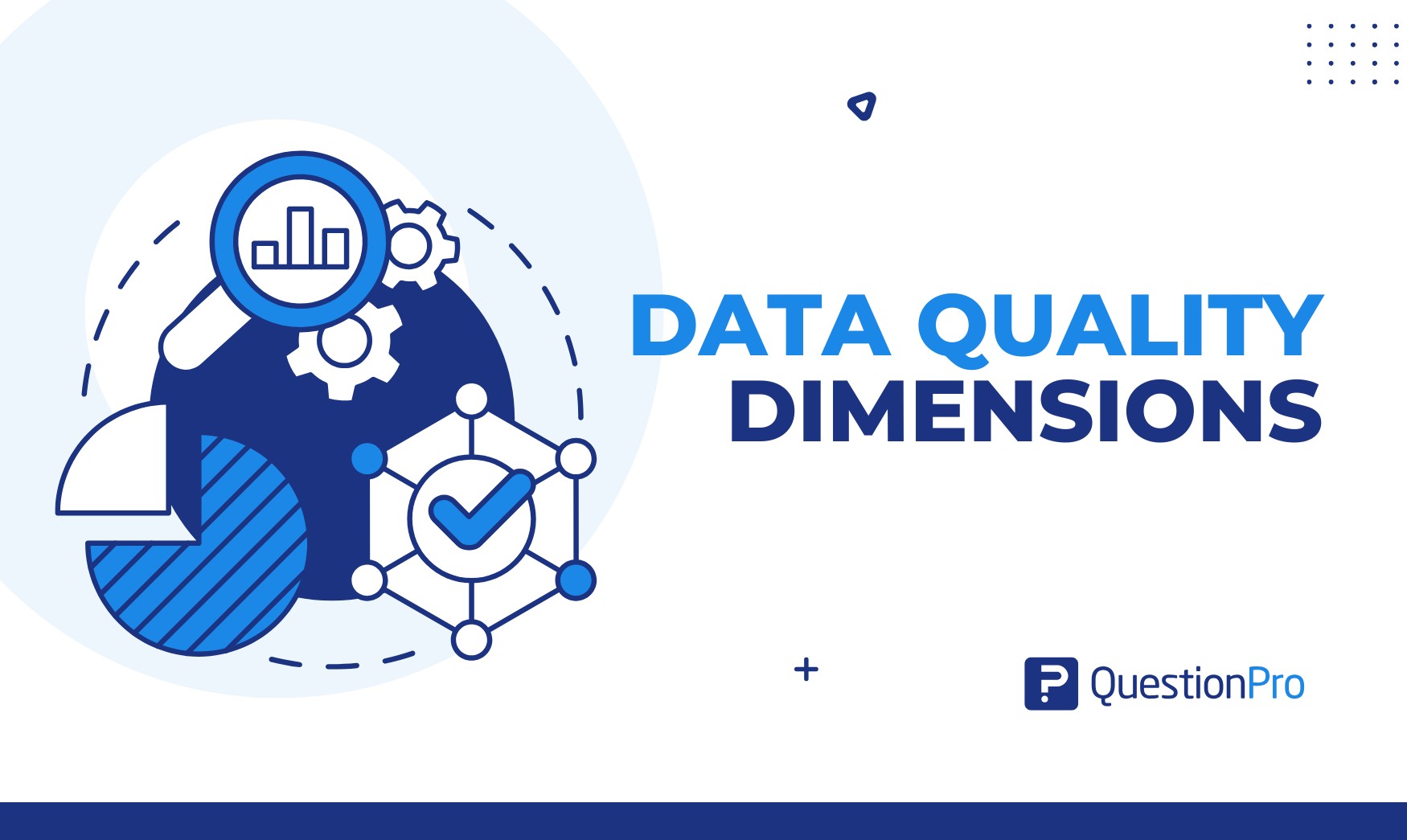
Data Quality Dimensions: What are They & How to Improve?
Dec 10, 2024
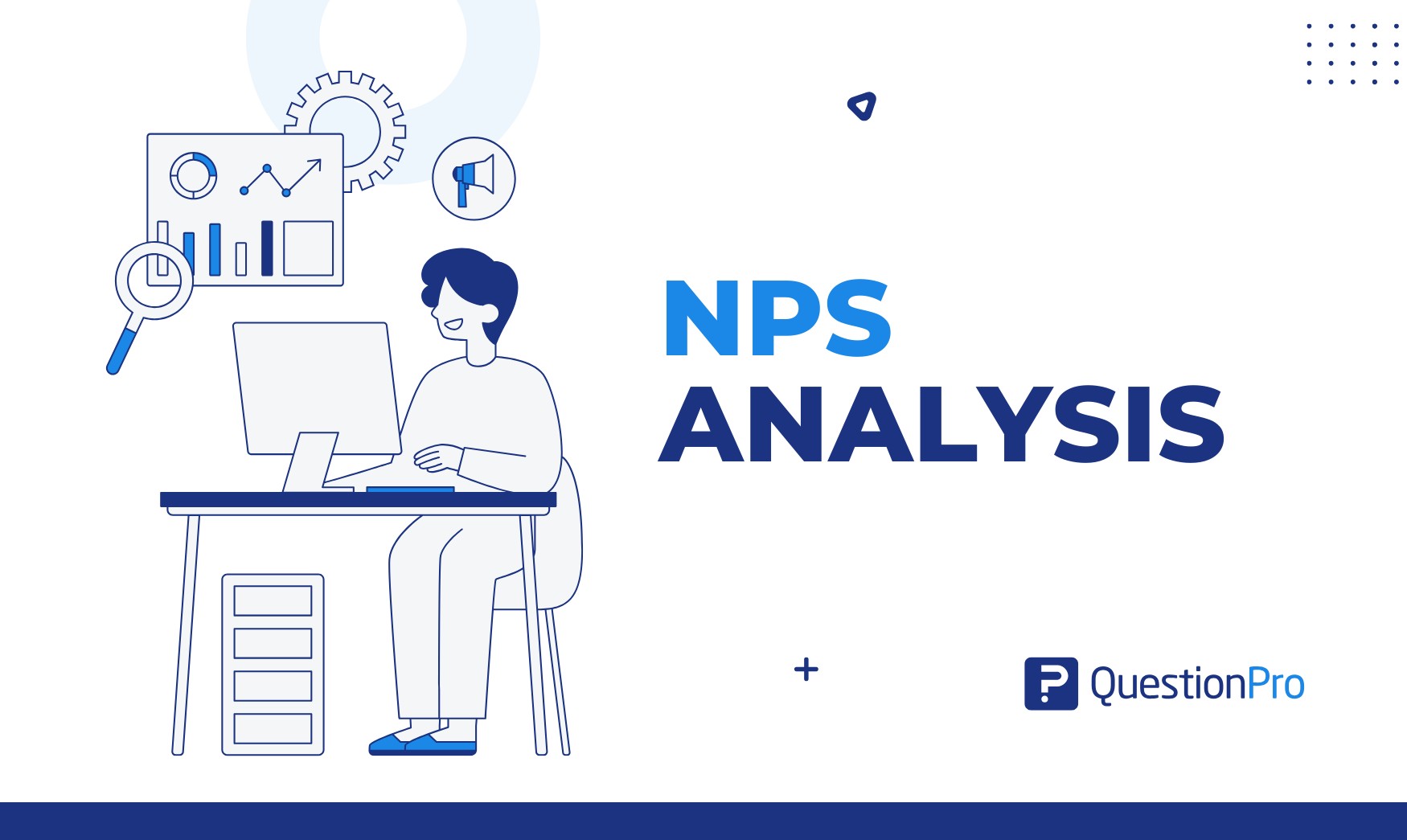
NPS Analysis: Boosting Customer Retention and Satisfaction
Dec 9, 2024
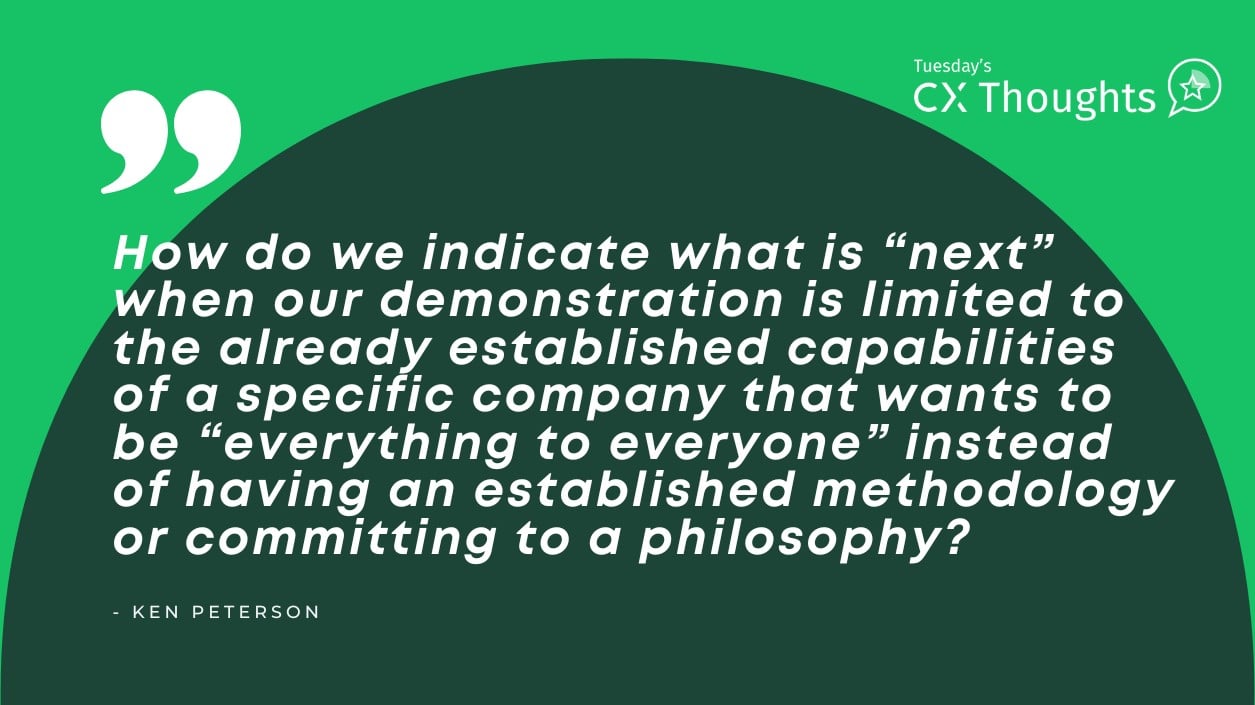
What Can We Expect Next? — Tuesday CX Thoughts
Dec 3, 2024
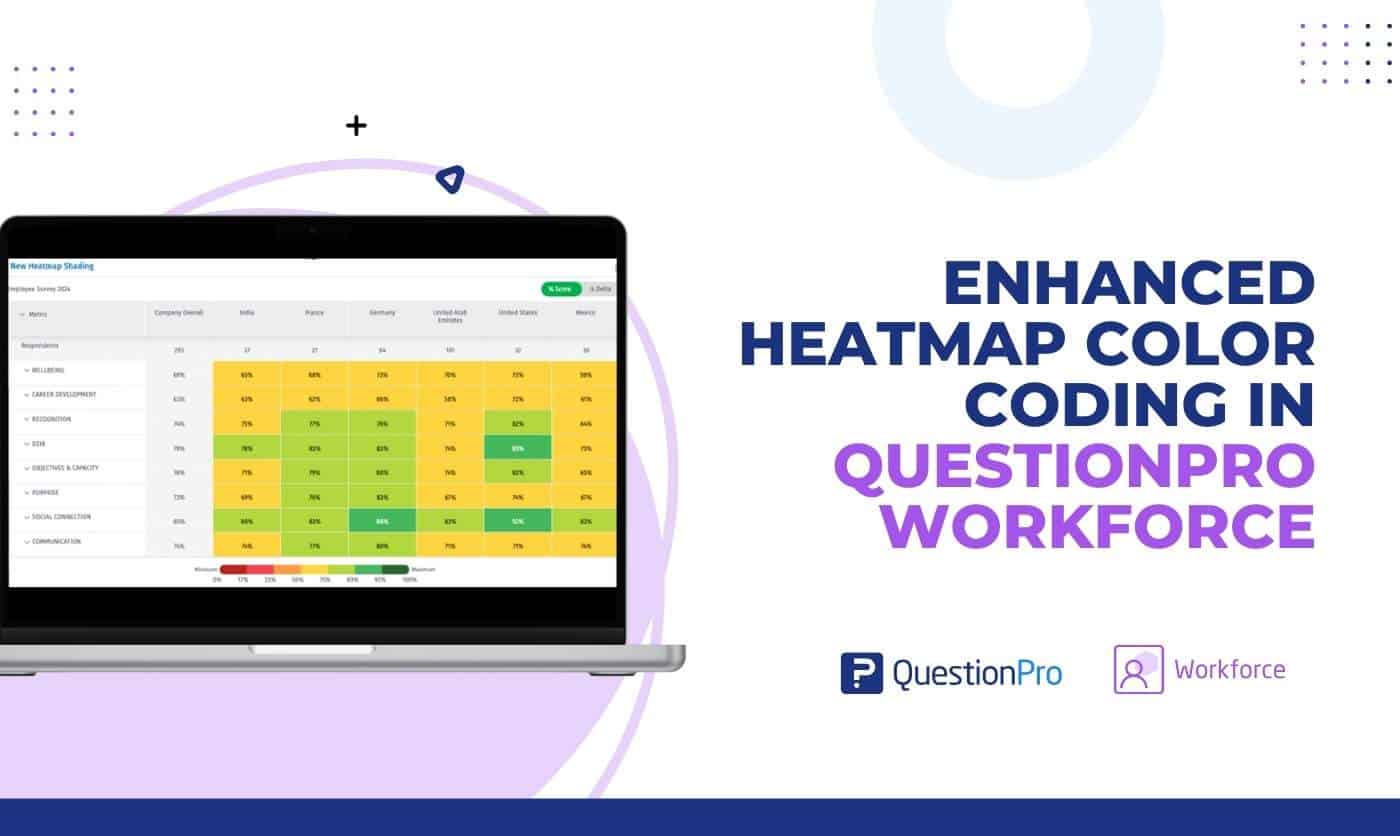
QuestionPro Workforce Turned Up the Heat 🔥
Dec 2, 2024
Other categories
- Academic Research
- Artificial Intelligence
- Assessments
- Brand Awareness
- Case Studies
- Communities
- Consumer Insights
- Customer effort score
- Customer Engagement
- Customer Experience
- Customer Loyalty
- Customer Research
- Customer Satisfaction
- Employee Benefits
- Employee Engagement
- Employee Retention
- Friday Five
- General Data Protection Regulation
- Insights Hub
- Life@QuestionPro
- Market Research
- Mobile diaries
- Mobile Surveys
- New Features
- Online Communities
- Question Types
- Questionnaire
- QuestionPro Products
- Release Notes
- Research Tools and Apps
- Revenue at Risk
- Survey Templates
- Training Tips
- Tuesday CX Thoughts (TCXT)
- Uncategorized
- What’s Coming Up
- Workforce Intelligence
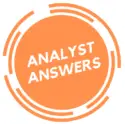
Data & Finance for Work & Life

Data Analysis: Types, Methods & Techniques (a Complete List)
( Updated Version )
While the term sounds intimidating, “data analysis” is nothing more than making sense of information in a table. It consists of filtering, sorting, grouping, and manipulating data tables with basic algebra and statistics.
In fact, you don’t need experience to understand the basics. You have already worked with data extensively in your life, and “analysis” is nothing more than a fancy word for good sense and basic logic.
Over time, people have intuitively categorized the best logical practices for treating data. These categories are what we call today types , methods , and techniques .
This article provides a comprehensive list of types, methods, and techniques, and explains the difference between them.
For a practical intro to data analysis (including types, methods, & techniques), check out our Intro to Data Analysis eBook for free.
Descriptive, Diagnostic, Predictive, & Prescriptive Analysis
If you Google “types of data analysis,” the first few results will explore descriptive , diagnostic , predictive , and prescriptive analysis. Why? Because these names are easy to understand and are used a lot in “the real world.”
Descriptive analysis is an informational method, diagnostic analysis explains “why” a phenomenon occurs, predictive analysis seeks to forecast the result of an action, and prescriptive analysis identifies solutions to a specific problem.
That said, these are only four branches of a larger analytical tree.
Good data analysts know how to position these four types within other analytical methods and tactics, allowing them to leverage strengths and weaknesses in each to uproot the most valuable insights.
Let’s explore the full analytical tree to understand how to appropriately assess and apply these four traditional types.
Tree diagram of Data Analysis Types, Methods, and Techniques
Here’s a picture to visualize the structure and hierarchy of data analysis types, methods, and techniques.
If it’s too small you can view the picture in a new tab . Open it to follow along!

Note: basic descriptive statistics such as mean , median , and mode , as well as standard deviation , are not shown because most people are already familiar with them. In the diagram, they would fall under the “descriptive” analysis type.
Tree Diagram Explained
The highest-level classification of data analysis is quantitative vs qualitative . Quantitative implies numbers while qualitative implies information other than numbers.
Quantitative data analysis then splits into mathematical analysis and artificial intelligence (AI) analysis . Mathematical types then branch into descriptive , diagnostic , predictive , and prescriptive .
Methods falling under mathematical analysis include clustering , classification , forecasting , and optimization . Qualitative data analysis methods include content analysis , narrative analysis , discourse analysis , framework analysis , and/or grounded theory .
Moreover, mathematical techniques include regression , Nïave Bayes , Simple Exponential Smoothing , cohorts , factors , linear discriminants , and more, whereas techniques falling under the AI type include artificial neural networks , decision trees , evolutionary programming , and fuzzy logic . Techniques under qualitative analysis include text analysis , coding , idea pattern analysis , and word frequency .
It’s a lot to remember! Don’t worry, once you understand the relationship and motive behind all these terms, it’ll be like riding a bike.
We’ll move down the list from top to bottom and I encourage you to open the tree diagram above in a new tab so you can follow along .
But first, let’s just address the elephant in the room: what’s the difference between methods and techniques anyway?
Difference between methods and techniques
Though often used interchangeably, methods ands techniques are not the same. By definition, methods are the process by which techniques are applied, and techniques are the practical application of those methods.
For example, consider driving. Methods include staying in your lane, stopping at a red light, and parking in a spot. Techniques include turning the steering wheel, braking, and pushing the gas pedal.
Data sets: observations and fields
It’s important to understand the basic structure of data tables to comprehend the rest of the article. A data set consists of one far-left column containing observations, then a series of columns containing the fields (aka “traits” or “characteristics”) that describe each observations. For example, imagine we want a data table for fruit. It might look like this:
Now let’s turn to types, methods, and techniques. Each heading below consists of a description, relative importance, the nature of data it explores, and the motivation for using it.
Quantitative Analysis
- It accounts for more than 50% of all data analysis and is by far the most widespread and well-known type of data analysis.
- As you have seen, it holds descriptive, diagnostic, predictive, and prescriptive methods, which in turn hold some of the most important techniques available today, such as clustering and forecasting.
- It can be broken down into mathematical and AI analysis.
- Importance : Very high . Quantitative analysis is a must for anyone interesting in becoming or improving as a data analyst.
- Nature of Data: data treated under quantitative analysis is, quite simply, quantitative. It encompasses all numeric data.
- Motive: to extract insights. (Note: we’re at the top of the pyramid, this gets more insightful as we move down.)
Qualitative Analysis
- It accounts for less than 30% of all data analysis and is common in social sciences .
- It can refer to the simple recognition of qualitative elements, which is not analytic in any way, but most often refers to methods that assign numeric values to non-numeric data for analysis.
- Because of this, some argue that it’s ultimately a quantitative type.
- Importance: Medium. In general, knowing qualitative data analysis is not common or even necessary for corporate roles. However, for researchers working in social sciences, its importance is very high .
- Nature of Data: data treated under qualitative analysis is non-numeric. However, as part of the analysis, analysts turn non-numeric data into numbers, at which point many argue it is no longer qualitative analysis.
- Motive: to extract insights. (This will be more important as we move down the pyramid.)
Mathematical Analysis
- Description: mathematical data analysis is a subtype of qualitative data analysis that designates methods and techniques based on statistics, algebra, and logical reasoning to extract insights. It stands in opposition to artificial intelligence analysis.
- Importance: Very High. The most widespread methods and techniques fall under mathematical analysis. In fact, it’s so common that many people use “quantitative” and “mathematical” analysis interchangeably.
- Nature of Data: numeric. By definition, all data under mathematical analysis are numbers.
- Motive: to extract measurable insights that can be used to act upon.
Artificial Intelligence & Machine Learning Analysis
- Description: artificial intelligence and machine learning analyses designate techniques based on the titular skills. They are not traditionally mathematical, but they are quantitative since they use numbers. Applications of AI & ML analysis techniques are developing, but they’re not yet mainstream enough to show promise across the field.
- Importance: Medium . As of today (September 2020), you don’t need to be fluent in AI & ML data analysis to be a great analyst. BUT, if it’s a field that interests you, learn it. Many believe that in 10 year’s time its importance will be very high .
- Nature of Data: numeric.
- Motive: to create calculations that build on themselves in order and extract insights without direct input from a human.
Descriptive Analysis
- Description: descriptive analysis is a subtype of mathematical data analysis that uses methods and techniques to provide information about the size, dispersion, groupings, and behavior of data sets. This may sounds complicated, but just think about mean, median, and mode: all three are types of descriptive analysis. They provide information about the data set. We’ll look at specific techniques below.
- Importance: Very high. Descriptive analysis is among the most commonly used data analyses in both corporations and research today.
- Nature of Data: the nature of data under descriptive statistics is sets. A set is simply a collection of numbers that behaves in predictable ways. Data reflects real life, and there are patterns everywhere to be found. Descriptive analysis describes those patterns.
- Motive: the motive behind descriptive analysis is to understand how numbers in a set group together, how far apart they are from each other, and how often they occur. As with most statistical analysis, the more data points there are, the easier it is to describe the set.
Diagnostic Analysis
- Description: diagnostic analysis answers the question “why did it happen?” It is an advanced type of mathematical data analysis that manipulates multiple techniques, but does not own any single one. Analysts engage in diagnostic analysis when they try to explain why.
- Importance: Very high. Diagnostics are probably the most important type of data analysis for people who don’t do analysis because they’re valuable to anyone who’s curious. They’re most common in corporations, as managers often only want to know the “why.”
- Nature of Data : data under diagnostic analysis are data sets. These sets in themselves are not enough under diagnostic analysis. Instead, the analyst must know what’s behind the numbers in order to explain “why.” That’s what makes diagnostics so challenging yet so valuable.
- Motive: the motive behind diagnostics is to diagnose — to understand why.
Predictive Analysis
- Description: predictive analysis uses past data to project future data. It’s very often one of the first kinds of analysis new researchers and corporate analysts use because it is intuitive. It is a subtype of the mathematical type of data analysis, and its three notable techniques are regression, moving average, and exponential smoothing.
- Importance: Very high. Predictive analysis is critical for any data analyst working in a corporate environment. Companies always want to know what the future will hold — especially for their revenue.
- Nature of Data: Because past and future imply time, predictive data always includes an element of time. Whether it’s minutes, hours, days, months, or years, we call this time series data . In fact, this data is so important that I’ll mention it twice so you don’t forget: predictive analysis uses time series data .
- Motive: the motive for investigating time series data with predictive analysis is to predict the future in the most analytical way possible.
Prescriptive Analysis
- Description: prescriptive analysis is a subtype of mathematical analysis that answers the question “what will happen if we do X?” It’s largely underestimated in the data analysis world because it requires diagnostic and descriptive analyses to be done before it even starts. More than simple predictive analysis, prescriptive analysis builds entire data models to show how a simple change could impact the ensemble.
- Importance: High. Prescriptive analysis is most common under the finance function in many companies. Financial analysts use it to build a financial model of the financial statements that show how that data will change given alternative inputs.
- Nature of Data: the nature of data in prescriptive analysis is data sets. These data sets contain patterns that respond differently to various inputs. Data that is useful for prescriptive analysis contains correlations between different variables. It’s through these correlations that we establish patterns and prescribe action on this basis. This analysis cannot be performed on data that exists in a vacuum — it must be viewed on the backdrop of the tangibles behind it.
- Motive: the motive for prescriptive analysis is to establish, with an acceptable degree of certainty, what results we can expect given a certain action. As you might expect, this necessitates that the analyst or researcher be aware of the world behind the data, not just the data itself.
Clustering Method
- Description: the clustering method groups data points together based on their relativeness closeness to further explore and treat them based on these groupings. There are two ways to group clusters: intuitively and statistically (or K-means).
- Importance: Very high. Though most corporate roles group clusters intuitively based on management criteria, a solid understanding of how to group them mathematically is an excellent descriptive and diagnostic approach to allow for prescriptive analysis thereafter.
- Nature of Data : the nature of data useful for clustering is sets with 1 or more data fields. While most people are used to looking at only two dimensions (x and y), clustering becomes more accurate the more fields there are.
- Motive: the motive for clustering is to understand how data sets group and to explore them further based on those groups.
- Here’s an example set:

Classification Method
- Description: the classification method aims to separate and group data points based on common characteristics . This can be done intuitively or statistically.
- Importance: High. While simple on the surface, classification can become quite complex. It’s very valuable in corporate and research environments, but can feel like its not worth the work. A good analyst can execute it quickly to deliver results.
- Nature of Data: the nature of data useful for classification is data sets. As we will see, it can be used on qualitative data as well as quantitative. This method requires knowledge of the substance behind the data, not just the numbers themselves.
- Motive: the motive for classification is group data not based on mathematical relationships (which would be clustering), but by predetermined outputs. This is why it’s less useful for diagnostic analysis, and more useful for prescriptive analysis.
Forecasting Method
- Description: the forecasting method uses time past series data to forecast the future.
- Importance: Very high. Forecasting falls under predictive analysis and is arguably the most common and most important method in the corporate world. It is less useful in research, which prefers to understand the known rather than speculate about the future.
- Nature of Data: data useful for forecasting is time series data, which, as we’ve noted, always includes a variable of time.
- Motive: the motive for the forecasting method is the same as that of prescriptive analysis: the confidently estimate future values.
Optimization Method
- Description: the optimization method maximized or minimizes values in a set given a set of criteria. It is arguably most common in prescriptive analysis. In mathematical terms, it is maximizing or minimizing a function given certain constraints.
- Importance: Very high. The idea of optimization applies to more analysis types than any other method. In fact, some argue that it is the fundamental driver behind data analysis. You would use it everywhere in research and in a corporation.
- Nature of Data: the nature of optimizable data is a data set of at least two points.
- Motive: the motive behind optimization is to achieve the best result possible given certain conditions.
Content Analysis Method
- Description: content analysis is a method of qualitative analysis that quantifies textual data to track themes across a document. It’s most common in academic fields and in social sciences, where written content is the subject of inquiry.
- Importance: High. In a corporate setting, content analysis as such is less common. If anything Nïave Bayes (a technique we’ll look at below) is the closest corporations come to text. However, it is of the utmost importance for researchers. If you’re a researcher, check out this article on content analysis .
- Nature of Data: data useful for content analysis is textual data.
- Motive: the motive behind content analysis is to understand themes expressed in a large text
Narrative Analysis Method
- Description: narrative analysis is a method of qualitative analysis that quantifies stories to trace themes in them. It’s differs from content analysis because it focuses on stories rather than research documents, and the techniques used are slightly different from those in content analysis (very nuances and outside the scope of this article).
- Importance: Low. Unless you are highly specialized in working with stories, narrative analysis rare.
- Nature of Data: the nature of the data useful for the narrative analysis method is narrative text.
- Motive: the motive for narrative analysis is to uncover hidden patterns in narrative text.
Discourse Analysis Method
- Description: the discourse analysis method falls under qualitative analysis and uses thematic coding to trace patterns in real-life discourse. That said, real-life discourse is oral, so it must first be transcribed into text.
- Importance: Low. Unless you are focused on understand real-world idea sharing in a research setting, this kind of analysis is less common than the others on this list.
- Nature of Data: the nature of data useful in discourse analysis is first audio files, then transcriptions of those audio files.
- Motive: the motive behind discourse analysis is to trace patterns of real-world discussions. (As a spooky sidenote, have you ever felt like your phone microphone was listening to you and making reading suggestions? If it was, the method was discourse analysis.)
Framework Analysis Method
- Description: the framework analysis method falls under qualitative analysis and uses similar thematic coding techniques to content analysis. However, where content analysis aims to discover themes, framework analysis starts with a framework and only considers elements that fall in its purview.
- Importance: Low. As with the other textual analysis methods, framework analysis is less common in corporate settings. Even in the world of research, only some use it. Strangely, it’s very common for legislative and political research.
- Nature of Data: the nature of data useful for framework analysis is textual.
- Motive: the motive behind framework analysis is to understand what themes and parts of a text match your search criteria.
Grounded Theory Method
- Description: the grounded theory method falls under qualitative analysis and uses thematic coding to build theories around those themes.
- Importance: Low. Like other qualitative analysis techniques, grounded theory is less common in the corporate world. Even among researchers, you would be hard pressed to find many using it. Though powerful, it’s simply too rare to spend time learning.
- Nature of Data: the nature of data useful in the grounded theory method is textual.
- Motive: the motive of grounded theory method is to establish a series of theories based on themes uncovered from a text.
Clustering Technique: K-Means
- Description: k-means is a clustering technique in which data points are grouped in clusters that have the closest means. Though not considered AI or ML, it inherently requires the use of supervised learning to reevaluate clusters as data points are added. Clustering techniques can be used in diagnostic, descriptive, & prescriptive data analyses.
- Importance: Very important. If you only take 3 things from this article, k-means clustering should be part of it. It is useful in any situation where n observations have multiple characteristics and we want to put them in groups.
- Nature of Data: the nature of data is at least one characteristic per observation, but the more the merrier.
- Motive: the motive for clustering techniques such as k-means is to group observations together and either understand or react to them.
Regression Technique
- Description: simple and multivariable regressions use either one independent variable or combination of multiple independent variables to calculate a correlation to a single dependent variable using constants. Regressions are almost synonymous with correlation today.
- Importance: Very high. Along with clustering, if you only take 3 things from this article, regression techniques should be part of it. They’re everywhere in corporate and research fields alike.
- Nature of Data: the nature of data used is regressions is data sets with “n” number of observations and as many variables as are reasonable. It’s important, however, to distinguish between time series data and regression data. You cannot use regressions or time series data without accounting for time. The easier way is to use techniques under the forecasting method.
- Motive: The motive behind regression techniques is to understand correlations between independent variable(s) and a dependent one.
Nïave Bayes Technique
- Description: Nïave Bayes is a classification technique that uses simple probability to classify items based previous classifications. In plain English, the formula would be “the chance that thing with trait x belongs to class c depends on (=) the overall chance of trait x belonging to class c, multiplied by the overall chance of class c, divided by the overall chance of getting trait x.” As a formula, it’s P(c|x) = P(x|c) * P(c) / P(x).
- Importance: High. Nïave Bayes is a very common, simplistic classification techniques because it’s effective with large data sets and it can be applied to any instant in which there is a class. Google, for example, might use it to group webpages into groups for certain search engine queries.
- Nature of Data: the nature of data for Nïave Bayes is at least one class and at least two traits in a data set.
- Motive: the motive behind Nïave Bayes is to classify observations based on previous data. It’s thus considered part of predictive analysis.
Cohorts Technique
- Description: cohorts technique is a type of clustering method used in behavioral sciences to separate users by common traits. As with clustering, it can be done intuitively or mathematically, the latter of which would simply be k-means.
- Importance: Very high. With regard to resembles k-means, the cohort technique is more of a high-level counterpart. In fact, most people are familiar with it as a part of Google Analytics. It’s most common in marketing departments in corporations, rather than in research.
- Nature of Data: the nature of cohort data is data sets in which users are the observation and other fields are used as defining traits for each cohort.
- Motive: the motive for cohort analysis techniques is to group similar users and analyze how you retain them and how the churn.
Factor Technique
- Description: the factor analysis technique is a way of grouping many traits into a single factor to expedite analysis. For example, factors can be used as traits for Nïave Bayes classifications instead of more general fields.
- Importance: High. While not commonly employed in corporations, factor analysis is hugely valuable. Good data analysts use it to simplify their projects and communicate them more clearly.
- Nature of Data: the nature of data useful in factor analysis techniques is data sets with a large number of fields on its observations.
- Motive: the motive for using factor analysis techniques is to reduce the number of fields in order to more quickly analyze and communicate findings.
Linear Discriminants Technique
- Description: linear discriminant analysis techniques are similar to regressions in that they use one or more independent variable to determine a dependent variable; however, the linear discriminant technique falls under a classifier method since it uses traits as independent variables and class as a dependent variable. In this way, it becomes a classifying method AND a predictive method.
- Importance: High. Though the analyst world speaks of and uses linear discriminants less commonly, it’s a highly valuable technique to keep in mind as you progress in data analysis.
- Nature of Data: the nature of data useful for the linear discriminant technique is data sets with many fields.
- Motive: the motive for using linear discriminants is to classify observations that would be otherwise too complex for simple techniques like Nïave Bayes.
Exponential Smoothing Technique
- Description: exponential smoothing is a technique falling under the forecasting method that uses a smoothing factor on prior data in order to predict future values. It can be linear or adjusted for seasonality. The basic principle behind exponential smoothing is to use a percent weight (value between 0 and 1 called alpha) on more recent values in a series and a smaller percent weight on less recent values. The formula is f(x) = current period value * alpha + previous period value * 1-alpha.
- Importance: High. Most analysts still use the moving average technique (covered next) for forecasting, though it is less efficient than exponential moving, because it’s easy to understand. However, good analysts will have exponential smoothing techniques in their pocket to increase the value of their forecasts.
- Nature of Data: the nature of data useful for exponential smoothing is time series data . Time series data has time as part of its fields .
- Motive: the motive for exponential smoothing is to forecast future values with a smoothing variable.
Moving Average Technique
- Description: the moving average technique falls under the forecasting method and uses an average of recent values to predict future ones. For example, to predict rainfall in April, you would take the average of rainfall from January to March. It’s simple, yet highly effective.
- Importance: Very high. While I’m personally not a huge fan of moving averages due to their simplistic nature and lack of consideration for seasonality, they’re the most common forecasting technique and therefore very important.
- Nature of Data: the nature of data useful for moving averages is time series data .
- Motive: the motive for moving averages is to predict future values is a simple, easy-to-communicate way.
Neural Networks Technique
- Description: neural networks are a highly complex artificial intelligence technique that replicate a human’s neural analysis through a series of hyper-rapid computations and comparisons that evolve in real time. This technique is so complex that an analyst must use computer programs to perform it.
- Importance: Medium. While the potential for neural networks is theoretically unlimited, it’s still little understood and therefore uncommon. You do not need to know it by any means in order to be a data analyst.
- Nature of Data: the nature of data useful for neural networks is data sets of astronomical size, meaning with 100s of 1000s of fields and the same number of row at a minimum .
- Motive: the motive for neural networks is to understand wildly complex phenomenon and data to thereafter act on it.
Decision Tree Technique
- Description: the decision tree technique uses artificial intelligence algorithms to rapidly calculate possible decision pathways and their outcomes on a real-time basis. It’s so complex that computer programs are needed to perform it.
- Importance: Medium. As with neural networks, decision trees with AI are too little understood and are therefore uncommon in corporate and research settings alike.
- Nature of Data: the nature of data useful for the decision tree technique is hierarchical data sets that show multiple optional fields for each preceding field.
- Motive: the motive for decision tree techniques is to compute the optimal choices to make in order to achieve a desired result.
Evolutionary Programming Technique
- Description: the evolutionary programming technique uses a series of neural networks, sees how well each one fits a desired outcome, and selects only the best to test and retest. It’s called evolutionary because is resembles the process of natural selection by weeding out weaker options.
- Importance: Medium. As with the other AI techniques, evolutionary programming just isn’t well-understood enough to be usable in many cases. It’s complexity also makes it hard to explain in corporate settings and difficult to defend in research settings.
- Nature of Data: the nature of data in evolutionary programming is data sets of neural networks, or data sets of data sets.
- Motive: the motive for using evolutionary programming is similar to decision trees: understanding the best possible option from complex data.
- Video example :
Fuzzy Logic Technique
- Description: fuzzy logic is a type of computing based on “approximate truths” rather than simple truths such as “true” and “false.” It is essentially two tiers of classification. For example, to say whether “Apples are good,” you need to first classify that “Good is x, y, z.” Only then can you say apples are good. Another way to see it helping a computer see truth like humans do: “definitely true, probably true, maybe true, probably false, definitely false.”
- Importance: Medium. Like the other AI techniques, fuzzy logic is uncommon in both research and corporate settings, which means it’s less important in today’s world.
- Nature of Data: the nature of fuzzy logic data is huge data tables that include other huge data tables with a hierarchy including multiple subfields for each preceding field.
- Motive: the motive of fuzzy logic to replicate human truth valuations in a computer is to model human decisions based on past data. The obvious possible application is marketing.
Text Analysis Technique
- Description: text analysis techniques fall under the qualitative data analysis type and use text to extract insights.
- Importance: Medium. Text analysis techniques, like all the qualitative analysis type, are most valuable for researchers.
- Nature of Data: the nature of data useful in text analysis is words.
- Motive: the motive for text analysis is to trace themes in a text across sets of very long documents, such as books.
Coding Technique
- Description: the coding technique is used in textual analysis to turn ideas into uniform phrases and analyze the number of times and the ways in which those ideas appear. For this reason, some consider it a quantitative technique as well. You can learn more about coding and the other qualitative techniques here .
- Importance: Very high. If you’re a researcher working in social sciences, coding is THE analysis techniques, and for good reason. It’s a great way to add rigor to analysis. That said, it’s less common in corporate settings.
- Nature of Data: the nature of data useful for coding is long text documents.
- Motive: the motive for coding is to make tracing ideas on paper more than an exercise of the mind by quantifying it and understanding is through descriptive methods.
Idea Pattern Technique
- Description: the idea pattern analysis technique fits into coding as the second step of the process. Once themes and ideas are coded, simple descriptive analysis tests may be run. Some people even cluster the ideas!
- Importance: Very high. If you’re a researcher, idea pattern analysis is as important as the coding itself.
- Nature of Data: the nature of data useful for idea pattern analysis is already coded themes.
- Motive: the motive for the idea pattern technique is to trace ideas in otherwise unmanageably-large documents.
Word Frequency Technique
- Description: word frequency is a qualitative technique that stands in opposition to coding and uses an inductive approach to locate specific words in a document in order to understand its relevance. Word frequency is essentially the descriptive analysis of qualitative data because it uses stats like mean, median, and mode to gather insights.
- Importance: High. As with the other qualitative approaches, word frequency is very important in social science research, but less so in corporate settings.
- Nature of Data: the nature of data useful for word frequency is long, informative documents.
- Motive: the motive for word frequency is to locate target words to determine the relevance of a document in question.
Types of data analysis in research
Types of data analysis in research methodology include every item discussed in this article. As a list, they are:
- Quantitative
- Qualitative
- Mathematical
- Machine Learning and AI
- Descriptive
- Prescriptive
- Classification
- Forecasting
- Optimization
- Grounded theory
- Artificial Neural Networks
- Decision Trees
- Evolutionary Programming
- Fuzzy Logic
- Text analysis
- Idea Pattern Analysis
- Word Frequency Analysis
- Nïave Bayes
- Exponential smoothing
- Moving average
- Linear discriminant
Types of data analysis in qualitative research
As a list, the types of data analysis in qualitative research are the following methods:
Types of data analysis in quantitative research
As a list, the types of data analysis in quantitative research are:
Data analysis methods
As a list, data analysis methods are:
- Content (qualitative)
- Narrative (qualitative)
- Discourse (qualitative)
- Framework (qualitative)
- Grounded theory (qualitative)
Quantitative data analysis methods
As a list, quantitative data analysis methods are:
Tabular View of Data Analysis Types, Methods, and Techniques
About the author.
Noah is the founder & Editor-in-Chief at AnalystAnswers. He is a transatlantic professional and entrepreneur with 5+ years of corporate finance and data analytics experience, as well as 3+ years in consumer financial products and business software. He started AnalystAnswers to provide aspiring professionals with accessible explanations of otherwise dense finance and data concepts. Noah believes everyone can benefit from an analytical mindset in growing digital world. When he's not busy at work, Noah likes to explore new European cities, exercise, and spend time with friends and family.
File available immediately.
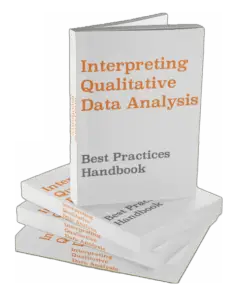
Notice: JavaScript is required for this content.
- Privacy Policy

Home » Data Analysis – Process, Methods and Types
Data Analysis – Process, Methods and Types
Table of Contents
Data analysis is the systematic process of inspecting, cleaning, transforming, and modeling data to uncover meaningful insights, support decision-making, and solve specific problems. In today’s data-driven world, data analysis is crucial for businesses, researchers, and policymakers to interpret trends, predict outcomes, and make informed decisions. This article delves into the data analysis process, commonly used methods, and the different types of data analysis.

Data Analysis
Data analysis involves the application of statistical, mathematical, and computational techniques to make sense of raw data. It transforms unorganized data into actionable information, often through visualizations, statistical summaries, or predictive models.
For example, analyzing sales data over time can help a retailer understand seasonal trends and forecast future demand.
Importance of Data Analysis
- Informed Decision-Making: Helps stakeholders make evidence-based choices.
- Problem Solving: Identifies patterns, relationships, and anomalies in data.
- Efficiency Improvement: Optimizes processes and operations through insights.
- Strategic Planning: Assists in setting realistic goals and forecasting outcomes.
Data Analysis Process
The process of data analysis typically follows a structured approach to ensure accuracy and reliability.
1. Define Objectives
Clearly articulate the research question or business problem you aim to address.
- Example: A company wants to analyze customer satisfaction to improve its services.

2. Data Collection
Gather relevant data from various sources, such as surveys, databases, or APIs.
- Example: Collect customer feedback through online surveys and customer service logs.
3. Data Cleaning
Prepare the data for analysis by removing errors, duplicates, and inconsistencies.
- Example: Handle missing values, correct typos, and standardize formats.
4. Data Exploration
Perform exploratory data analysis (EDA) to understand data patterns, distributions, and relationships.
- Example: Use summary statistics and visualizations like histograms or scatter plots.
5. Data Transformation
Transform raw data into a usable format by scaling, encoding, or aggregating.
- Example: Convert categorical data into numerical values for machine learning algorithms.
6. Analysis and Interpretation
Apply appropriate methods or models to analyze the data and extract insights.
- Example: Use regression analysis to predict customer churn rates.
7. Reporting and Visualization
Present findings in a clear and actionable format using dashboards, charts, or reports.
- Example: Create a dashboard summarizing customer satisfaction scores by region.
8. Decision-Making and Implementation
Use the insights to make recommendations or implement strategies.
- Example: Launch targeted marketing campaigns based on customer preferences.
Methods of Data Analysis
1. statistical methods.
- Descriptive Statistics: Summarizes data using measures like mean, median, and standard deviation.
- Inferential Statistics: Draws conclusions or predictions from sample data using techniques like hypothesis testing or confidence intervals.
2. Data Mining
Data mining involves discovering patterns, correlations, and anomalies in large datasets.
- Example: Identifying purchasing patterns in retail through association rules.
3. Machine Learning
Applies algorithms to build predictive models and automate decision-making.
- Example: Using supervised learning to classify email spam.
4. Text Analysis
Analyzes textual data to extract insights, often used in sentiment analysis or topic modeling.
- Example: Analyzing customer reviews to understand product sentiment.
5. Time-Series Analysis
Focuses on analyzing data points collected over time to identify trends and patterns.
- Example: Forecasting stock prices based on historical data.
6. Data Visualization
Transforms data into visual representations like charts, graphs, and heatmaps to make findings comprehensible.
- Example: Using bar charts to compare monthly sales performance.
7. Predictive Analytics
Uses statistical models and machine learning to forecast future outcomes based on historical data.
- Example: Predicting the likelihood of equipment failure in a manufacturing plant.
8. Diagnostic Analysis
Focuses on identifying causes of observed patterns or trends in data.
- Example: Investigating why sales dropped in a particular quarter.
Types of Data Analysis
1. descriptive analysis.
- Purpose: Summarizes raw data to provide insights into past trends and performance.
- Example: Analyzing average customer spending per month.
2. Exploratory Analysis
- Purpose: Identifies patterns, relationships, or hypotheses for further study.
- Example: Exploring correlations between advertising spend and sales.
3. Inferential Analysis
- Purpose: Draws conclusions or makes predictions about a population based on sample data.
- Example: Estimating national voter preferences using survey data.
4. Diagnostic Analysis
- Purpose: Examines the reasons behind observed outcomes or trends.
- Example: Investigating why website traffic decreased after a redesign.
5. Predictive Analysis
- Purpose: Forecasts future outcomes based on historical data.
- Example: Predicting customer churn using machine learning algorithms.
6. Prescriptive Analysis
- Purpose: Recommends actions based on data insights and predictive models.
- Example: Suggesting the best marketing channels to maximize ROI.
Tools for Data Analysis
1. programming languages.
- Python: Popular for data manipulation, analysis, and machine learning (e.g., Pandas, NumPy, Scikit-learn).
- R: Ideal for statistical computing and visualization.
2. Data Visualization Tools
- Tableau: Creates interactive dashboards and visualizations.
- Power BI: Microsoft’s tool for business intelligence and reporting.
3. Statistical Software
- SPSS: Used for statistical analysis in social sciences.
- SAS: Advanced analytics, data management, and predictive modeling tool.
4. Big Data Platforms
- Hadoop: Framework for processing large-scale datasets.
- Apache Spark: Fast data processing engine for big data analytics.
5. Spreadsheet Tools
- Microsoft Excel: Widely used for basic data analysis and visualization.
- Google Sheets: Collaborative online spreadsheet tool.
Challenges in Data Analysis
- Data Quality Issues: Missing, inconsistent, or inaccurate data can compromise results.
- Scalability: Analyzing large datasets requires advanced tools and computing power.
- Bias in Data: Skewed datasets can lead to misleading conclusions.
- Complexity: Choosing the appropriate analysis methods and models can be challenging.
Applications of Data Analysis
- Business: Improving customer experience through sales and marketing analytics.
- Healthcare: Analyzing patient data to improve treatment outcomes.
- Education: Evaluating student performance and designing effective teaching strategies.
- Finance: Detecting fraudulent transactions using predictive models.
- Social Science: Understanding societal trends through demographic analysis.
Data analysis is an essential process for transforming raw data into actionable insights. By understanding the process, methods, and types of data analysis, researchers and professionals can effectively tackle complex problems, uncover trends, and make data-driven decisions. With advancements in tools and technology, the scope and impact of data analysis continue to expand, shaping the future of industries and research.
- McKinney, W. (2017). Python for Data Analysis: Data Wrangling with Pandas, NumPy, and IPython . O’Reilly Media.
- Han, J., Pei, J., & Kamber, M. (2011). Data Mining: Concepts and Techniques . Morgan Kaufmann.
- Provost, F., & Fawcett, T. (2013). Data Science for Business: What You Need to Know About Data Mining and Data-Analytic Thinking . O’Reilly Media.
- Montgomery, D. C., & Runger, G. C. (2018). Applied Statistics and Probability for Engineers . Wiley.
- Tableau Public (2023). Creating Data Visualizations and Dashboards . Retrieved from https://www.tableau.com.
About the author
Muhammad Hassan
Researcher, Academic Writer, Web developer
You may also like

Research Report – Example, Writing Guide and...

Problem Statement – Writing Guide, Examples and...

Data Verification – Process, Types and Examples

Grounded Theory – Methods, Examples and Guide

Content Analysis – Methods, Types and Examples

Regression Analysis – Methods, Types and Examples
From ANOVA to regression: 10 key statistical analysis methods explained
Last updated
24 October 2024
Reviewed by
Miroslav Damyanov
Every action we take generates data. When you stream a video, browse a website, or even make a purchase, valuable data is created. However, without statistical analysis, the potential of this information remains untapped.
Understanding how different statistical analysis methods work can help you make the right choice. Each is applicable to a certain situation, data type, and goal.
- What is statistical analysis?
Statistical analysis is the process of collecting, organizing, and interpreting data. The goal is to identify trends and relationships. These insights help analysts forecast outcomes and make strategic business decisions.
This type of analysis can apply to multiple business functions and industries, including the following:
Finance : helps companies assess investment risks and performance
Marketing : enables marketers to identify customer behavior patterns, segment markets, and measure the effectiveness of advertising campaigns
Operations: helps streamline process optimization and reduce waste
Human resources : helps track employee performance trends or analyze turnover rates
Product development : helps with feature prioritization, evaluating A/B test results, and improving product iterations based on user data
Scientific research: supports hypothesis testing, experiment validation, and the identification of significant relations in data
Government: informs public policy decisions, such as understanding population demographics or analyzing inflation
With high-quality statistical analysis, businesses can base their decisions on data-driven insights rather than assumptions. This helps build more effective strategies and ultimately improves the bottom line.
- Importance of statistical analysis
Statistical analysis is an integral part of working with data. Implementing it at different stages of operations or research helps you gain insights that prevent costly errors.
Here are the key benefits of statistical analysis:
Informed decision-making
Statistical analysis allows businesses to base their decisions on solid data rather than assumptions.
By collecting and interpreting data, decision-makers can evaluate the potential outcomes of their strategies before they implement them. This approach reduces risks and increases the chances of success.
Understanding relationships and trends
In many complex environments, the key to insights is understanding relationships between different variables. Statistical methods such as regression or factor analysis help uncover these relationships.
Uncovering correlations through statistical methods can pave the way for breakthroughs in fields like medicine, but the true impact lies in identifying and validating cause-effect relationships. By distinguishing between simple associations and meaningful patterns, statistical analysis helps guide critical decisions, such as developing potentially life-saving treatments.
Predicting future outcomes
Statistical analysis, particularly predictive analysis and time series analysis, provides businesses with tools to forecast events based on historical data.
These forecasts help organizations prepare for future challenges (such as fluctuations in demand, market trends, or operational bottlenecks). Being able to predict outcomes allows for better resource allocation and risk mitigation.
Improving efficiency and reducing waste
Using statistical analysis can lead to improved efficiency in areas where waste occurs. In operations, this can result in streamlining processes.
For example, manufacturers can use causal analysis to identify the factors contributing to defective products and then implement targeted improvements to eliminate the causes.
Enhancing accuracy in research
In scientific research, statistical methods ensure accurate results by validating hypotheses and analyzing experimental data.
Methods such as regression analysis and ANOVA (analysis of variance) allow researchers to draw conclusions from experiments by examining relationships between variables and identifying key factors that influence outcomes.
Without statistical analysis, research findings may not be reliable. This could result in teams drawing incorrect conclusions and forming strategies that cost more than they’re worth.
Validating business assumptions
When businesses make assumptions about customer preferences, market conditions, or operational outcomes, statistical analysis can validate them.
For example, hypothesis testing can provide a framework to either confirm or reject an assumption. With these results at hand, businesses reduce the likelihood of pursuing incorrect strategies and improve their overall performance.
- Types of statistical analysis
The two main types of statistical analysis are descriptive and inferential. However, there are also other types. Here’s a short breakdown:
Descriptive analysis
Descriptive analysis focuses on summarizing and presenting data in a clear and understandable way. You can do this with simple tools like graphs and charts.
This type of statistical analysis helps break down large datasets into smaller, digestible pieces. This is usually done by calculating averages, frequencies, and ranges. The goal is to present the data in an orderly fashion and answer the question, “What happened?”
Businesses can use descriptive analysis to evaluate customer demographics or sales trends. A visual breakdown of complex data is often useful enough for people to come to useful conclusions.
Diagnostic statistics
This analysis is used to determine the cause of a particular outcome or behavior by examining relationships between variables. It answers the question, “Why did this happen?”
This approach often involves identifying anomalies or trends in data to understand underlying issues.
Inferential analysis
Inferential analysis involves drawing conclusions about a larger population based on a sample of data. It helps predict trends and test hypotheses by accounting for uncertainty and potential errors in the data.
For example, a marketing team can arrive at a conclusion about their potential audience’s demographics by analyzing their existing customer base. Another example is vaccine trials, which allow researchers to come to conclusions about side effects based on how the trial group reacts.
Predictive analysis
Predictive analysis uses historical data to forecast future outcomes. It answers the question, “What might happen in the future?”
For example, a business owner can predict future customer behavior by analyzing their past interactions with the company. Meanwhile, marketers can anticipate which products are likely to succeed based on past sales data.
This type of analysis requires the implementation of complex techniques to ensure the expected results. These results are still educated guesses—not error-free conclusions.
Prescriptive analysis
Prescriptive analysis goes beyond predicting outcomes. It suggests actionable steps to achieve desired results.
This type of statistical analysis combines data, algorithms, and business rules to recommend actual strategies. It often uses optimization techniques to suggest the best course of action in a given scenario, answering the question, “What should we do next?”
For example, in supply chain management, prescriptive analysis helps optimize inventory levels by providing specific recommendations based on forecasts. A bank can use this analysis to predict loan defaults based on economic trends and adjust lending policies accordingly.
Exploratory data analysis
Exploratory data analysis (EDA) allows you to investigate datasets to discover patterns or anomalies without predefined hypotheses. This approach can summarize a dataset’s main characteristics, often using visual methods.
EDA is particularly useful for uncovering new insights that weren’t anticipated during initial data collection .
Causal analysis
Causal analysis seeks to identify cause-and-effect relationships between variables. It helps determine why certain events happen, often employing techniques such as experiments or quasi-experimental designs to establish causality.
Understanding the “why” of specific events can help design accurate proactive and reactive strategies.
For example, in marketing, causal analysis can be applied to understand the impact of a new advertising campaign on sales.
Bayesian statistics
This approach incorporates prior knowledge or beliefs into the statistical analysis. It involves updating the probability of a hypothesis as more evidence becomes available.
- Statistical analysis methods
Depending on your industry, needs, and budget, you can implement different statistical analysis methods. Here are some of the most common techniques:
A t-test helps determine if there’s a significant difference between the means of two groups. It works well when you want to compare the average performance of two groups under different conditions.
There are different types of t-tests, including independent or dependent.
T-tests are often used in research experiments and quality control processes. For example, they work well in drug testing when one group receives a real drug and another receives a placebo. If the group that received a real drug shows significant improvements, a t-test helps determine if the improvement is real or chance-related.
2. Chi-square tests
Chi-square tests examine the relationship between categorical variables. They compare observed results with expected results. The goal is to understand if the difference between the two is due to chance or the relationship between the variables.
For instance, a company might use a chi-square test to analyze whether customer preferences for a product differ by region.
It’s particularly useful in market research , where businesses analyze responses to surveys .
ANOVA, which stands for analysis of variance, compares the means of three or more groups to determine if there are statistically significant differences among them.
Unlike t-tests, which are limited to two groups, ANOVA is ideal when comparing multiple groups at once.
One-way ANOVA: analysis with one independent variable and one dependent variable
Two-way ANOVA: analysis with two independent variables
Multivariate ANOVA (MANOVA): analysis with more than two independent variables
Businesses often use ANOVA to compare product performance across different markets and evaluate customer satisfaction across various demographics. The method is also common in experimental research, where multiple groups are exposed to different conditions.
4. Regression analysis
Regression analysis examines the relationship between one dependent variable and one or more independent variables. It helps businesses and researchers predict outcomes and understand which factors influence results the most.
This method determines a best-fit line and allows the researcher to observe how the data is distributed around this line.
It helps economists with asset valuations and predictions. It can also help marketers determine how variables like advertising affect sales.
A company might use regression analysis to forecast future sales based on marketing spend, product price, and customer demographics.
6. Time series analysis
Time series analysis evaluates data points collected over time to identify trends. An analyst records data points at equal intervals over a certain period instead of doing it randomly.
This method can help businesses and researchers forecast future outcomes based on historical data. For example, retailers might use time series analysis to plan inventory around holiday shopping trends, while financial institutions rely on it to track stock market trends. An energy company can use it to evaluate consumption trends and streamline the production schedule.
7. Survival analysis
Survival analysis focuses on time-to-event data, such as the time it takes for a machine to break down or for a customer to churn. It looks at a variable with a start time and end time. The time between them is the focus of the analysis.
This method is highly useful in medical research—for example, when studying the time between the beginning of a patient’s cancer remission and relapse. It can help doctors understand which treatments have desired or unexpected effects.
This analysis also has important applications in business. For example, companies use survival analysis to predict customer retention , product lifespan, or time until product failure.
8. Factor analysis
Factor analysis (FA) reduces large sets of variables into fewer components. It’s useful when dealing with complex datasets because it helps identify underlying structures and simplify data interpretation. This analysis is great for extracting maximum common variance from all necessary variables and turning them into a single score.
For example, in market research, businesses use factor analysis to group customer responses into broad categories. This helps reveal hidden patterns in consumer behavior .
It’s also helpful in product development, where it can use survey data to identify which product features are most important to customers.
9. Cluster analysis
Cluster analysis groups objects or individuals based on their similarities. This technique works great for customer segmentation, where businesses group customers based on common factors (such as purchasing behavior, demographics, and location).
Distinct clusters help companies tailor marketing strategies and develop personalized services. In education, this analysis can help identify groups of students who require additional assistance based on their achievement data. In medicine, it can help identify patients with similar symptoms to create targeted treatment plans.
10. Principal component analysis
Principal component analysis (PCA) is a dimensionality-reduction technique that simplifies large datasets by converting them into fewer components. It helps remove similar data from the line of comparison without affecting the data’s quality.
PCA is widely used in fields like finance, marketing, and genetics because it helps handle large datasets with many variables. For example, marketers can use PCA to identify which factors most influence customer buying decisions.
- How to choose the right statistical analysis method
Since numerous statistical analysis methods exist, choosing the right one for your needs may be complicated. While all of them can be applicable to the same situation, understanding where to start can save time and money.
Define your objective
Before choosing any statistical method, clearly define the objective of your analysis. What do you want to find out? Are you looking to compare groups, predict outcomes, or identify relationships between variables?
For example, if your goal is to compare averages between two groups, you can use a t-test. If you want to understand the effect of multiple factors on a single outcome, regression analysis could be the right choice for you.
Identify your data type
Data can be categorical (like yes/no or product types) or numerical (like sales figures or temperature readings).
For example, if you’re analyzing the relationship between two categorical variables, you may need a chi-square test. If you’re working with numerical data and need to predict future outcomes, you could use a time series analysis.
Evaluate the number of variables
The number of variables involved in your analysis influences the method you should choose. If you’re working with one dependent variable and one or more independent variables, regression analysis or ANOVA may be appropriate.
If you’re handling multiple variables, factor analysis or PCA can help simplify your dataset.
Determine sample size and data availability
Consider the assumptions of each method.
Each statistical method has its own set of assumptions, such as the distribution of the data or the relationship between variables.
For example, ANOVA assumes that the groups being compared have similar variances, while regression assumes a linear relationship between independent and dependent variables .
Understand if observations are paired or unpaired
When choosing a statistical test, you need to figure out if the data is paired or unpaired.
Paired data : the same subjects are measured more than once, like before and after a treatment or when using different methods.
Unpaired data: each group has different subjects.
For example, if you’re comparing the average scores of two groups, use a paired t-test for paired data and an independent t-test for unpaired data.
- Making the most of key statistical analysis methods
Each statistical analysis method is designed to simplify the process of gaining insights from a specific dataset. Understanding which data you need to analyze and which results you want to see can help you choose the right method.
With a comprehensive approach to analytics, you can maximize the benefits of insights and streamline decision-making. This isn’t just applicable in research and science. Businesses across multiple industries can reap significant benefits from well-structured statistical analysis.
Should you be using a customer insights hub?
Do you want to discover previous research faster?
Do you share your research findings with others?
Do you analyze research data?
Start for free today, add your research, and get to key insights faster
Editor’s picks
Last updated: 9 November 2024
Last updated: 11 January 2024
Last updated: 14 February 2024
Last updated: 27 January 2024
Last updated: 17 January 2024
Last updated: 14 November 2023
Last updated: 20 January 2024
Last updated: 19 November 2023
Last updated: 5 February 2024
Last updated: 25 November 2024
Last updated: 25 November 2023
Last updated: 13 May 2024
Latest articles
Related topics, a whole new way to understand your customer is here, log in or sign up.
Get started for free

13 Essential Data Analysis Methods and Techniques to Unlock Success
Data Analysis Methods and Techniques: Unlocking Insights for Success
In today’s data-driven world, understanding and leveraging data analysis methods and techniques is crucial for businesses and researchers alike. Data analysis methods and techniques transforms raw data into meaningful insights, guiding decision-making and strategic planning. This comprehensive guide explores the various data analysis methods and techniques, their applications, and how they can propel your organization forward.
Table of Contents
Introduction to data analysis methods and techniques.
Data analysis methods and techniques involve the systematic application of statistical and logical procedures to describe, summarize, and compare data. By employing various methods and tools, analysts can uncover patterns, test hypotheses, and drive evidence-based decisions. Whether it’s improving business operations or advancing academic research, data analysis methods and techniques are the cornerstone of informed action.
Importance of Data Analysis Methods and Techniques
Implementing the correct data analysis methods is vital for:
- Accurate Insights : Ensuring data is interpreted correctly to make sound decisions.
- Efficiency : Streamlining the analysis process by using appropriate techniques.
- Strategic Planning : Guiding long-term strategies with reliable data.
- Competitive Advantage : Gaining an edge by understanding market trends and customer behaviors.
For more on why data analysis is crucial for businesses, read our article on Why Data Analysis is Important for Businesses .
Quantitative Data Analysis Methods and Techniques
Quantitative data analysis involves numerical data that can be measured and compared mathematically. Here are some fundamental methods:
3.1 Descriptive Statistics in Data Analysis
Descriptive statistics summarize data features through measures like mean, median, mode, and standard deviation.
- Example : Calculating the average sales revenue over the past year to understand business performance.
- Case Study: A Descriptive Case Study on Technology’s Impact in Elementary Classrooms .
3.2 Inferential Statistics
Inferential statistics allow analysts to make predictions or inferences about a population based on a sample.
- Example : Estimating customer satisfaction levels by surveying a sample group.
- Case Study: Influenza Vaccine Effectiveness in Canada: A 2007-2008 Study on Cross-Protection Against Diverse Strains
3.3 Regression Analysis Techniques
Regression analysis examines the relationship between dependent and independent variables.
- Application : Predicting sales based on advertising spend.
- Case Study: Analyzing the Impact of Advertising on Sales: A Regression Analysis Case Study from Benetton
Learn more : Our guide on SQL for Data Analysis explores how to use SQL in regression analysis.
3.4 Factor Analysis
Factor analysis reduces data by identifying underlying factors that explain patterns among variables.
- Use Case : Determining key factors that influence customer loyalty.
- Case Study: Identifying Shopping Habits in Tehran: A Factor Analysis Study by Ali Akbar Jowkar and Team
3.5 Time Series Analysis
Time series analysis studies data points collected or recorded at specific time intervals.
- Application : Forecasting stock prices or sales trends over time.
- Case Study: Optimizing Jute Yarn Demand Forecasting in Bangladesh: A Time Series Analysis Study
Qualitative Data Analysis Methods and Techniques
Qualitative data analysis methods and techniques focuses on understanding the content of non-numerical data like text, images, or audio.
Content Analysis
Content analysis systematically examines communication materials to identify patterns, themes, or biases.
For example, analyzing social media posts to gauge public sentiment about a new product launch.
Thematic Analysis
Thematic analysis identifies patterns or themes within qualitative data, providing insights into the underlying messages.
A researcher might use thematic analysis to explore common themes in interview transcripts.
Narrative Analysis
Narrative analysis looks at the stories individuals tell, examining the structure and content to understand how people make sense of experiences.
This method could be used in psychology to understand patient experiences through their personal narratives.
Discourse Analysis
Discourse analysis studies written or spoken language in relation to its social context, examining how language is used to achieve certain effects.
An example is analyzing political speeches to understand how language influences public opinion.
Grounded Theory
Grounded theory involves developing theories based on data systematically gathered and analyzed. It allows the theory to emerge from the data.
Researchers might use grounded theory to develop new models of consumer behavior.
Advanced Techniques in Data Analysis
With the increasing complexity of data, advanced methods are often required.
Machine Learning and AI
Machine learning algorithms enable computers to learn from data and make predictions or decisions without being explicitly programmed.
Applications include recommendation systems, fraud detection, and predictive analytics.
For an in-depth look, read our article on How to Use AI in Data Analysis .
Big Data Analysis Methods
Big data analysis handles extremely large and complex data sets that traditional data processing software can’t manage.
It’s used in various fields like finance, healthcare, and marketing to uncover hidden patterns and correlations.
Explore Big Data Analysis Methods for more information.
Monte Carlo Simulation
Monte Carlo simulation uses randomness to solve problems that might be deterministic in principle. It models the probability of different outcomes in processes that cannot easily be predicted.
This method is often used in financial risk assessment and project management.
Case Study: A case study using Monte Carlo simulation for risk analysis .
Choosing the Right Data Analysis Method
Selecting the appropriate data analysis method depends on:
- The Research Question : Define what you want to find out.
- Type of Data : Determine whether your data is quantitative, qualitative, or a mix.
- Resources Available : Consider the tools and expertise at your disposal.
- Desired Outcome : Are you looking to describe, predict, or explain phenomena?
Understanding these factors will guide you in choosing methods that are most effective for your specific needs.
FAQs on Data Analysis Methods
1. What are data analysis methods in research?
Data analysis methods in research are systematic approaches used to collect, process, and interpret data to answer research questions or test hypotheses.
2. How do qualitative and quantitative data analysis differ?
Quantitative analysis focuses on numerical data and statistical techniques, while qualitative analysis deals with non-numerical data and explores themes and patterns.
3. Why is regression analysis important?
Regression analysis is important because it helps identify relationships between variables, allowing for predictions and informed decision-making.
4. What is the role of big data analysis methods?
Big data analysis methods enable the processing of large, complex data sets to uncover patterns, trends, and associations that might be missed with smaller data sets.
5. How do I choose the right data analysis technique?
Consider your research objectives, the type of data you have, and what you aim to achieve with the analysis. This will help you select the most suitable method.
Data analysis methods and techniques are indispensable tools in today’s information-rich environment. By applying the right methods, organizations and researchers can transform raw data into valuable insights that drive strategic decisions and foster success.
To further enhance your data analysis capabilities, consider exploring our resources on:
- Data Analysis Tools: Top Solutions (October 2024)
- Data Analysis Types: A Comprehensive Guide .
Embracing the power of data analysis not only equips you with the knowledge to make informed decisions but also positions you ahead in a competitive landscape.
Ready to take your data analysis to the next level? Discover the 7 Top Data Analytics Tools in 2024 (May 2024) and revolutionize the way you interpret data.
Related Posts
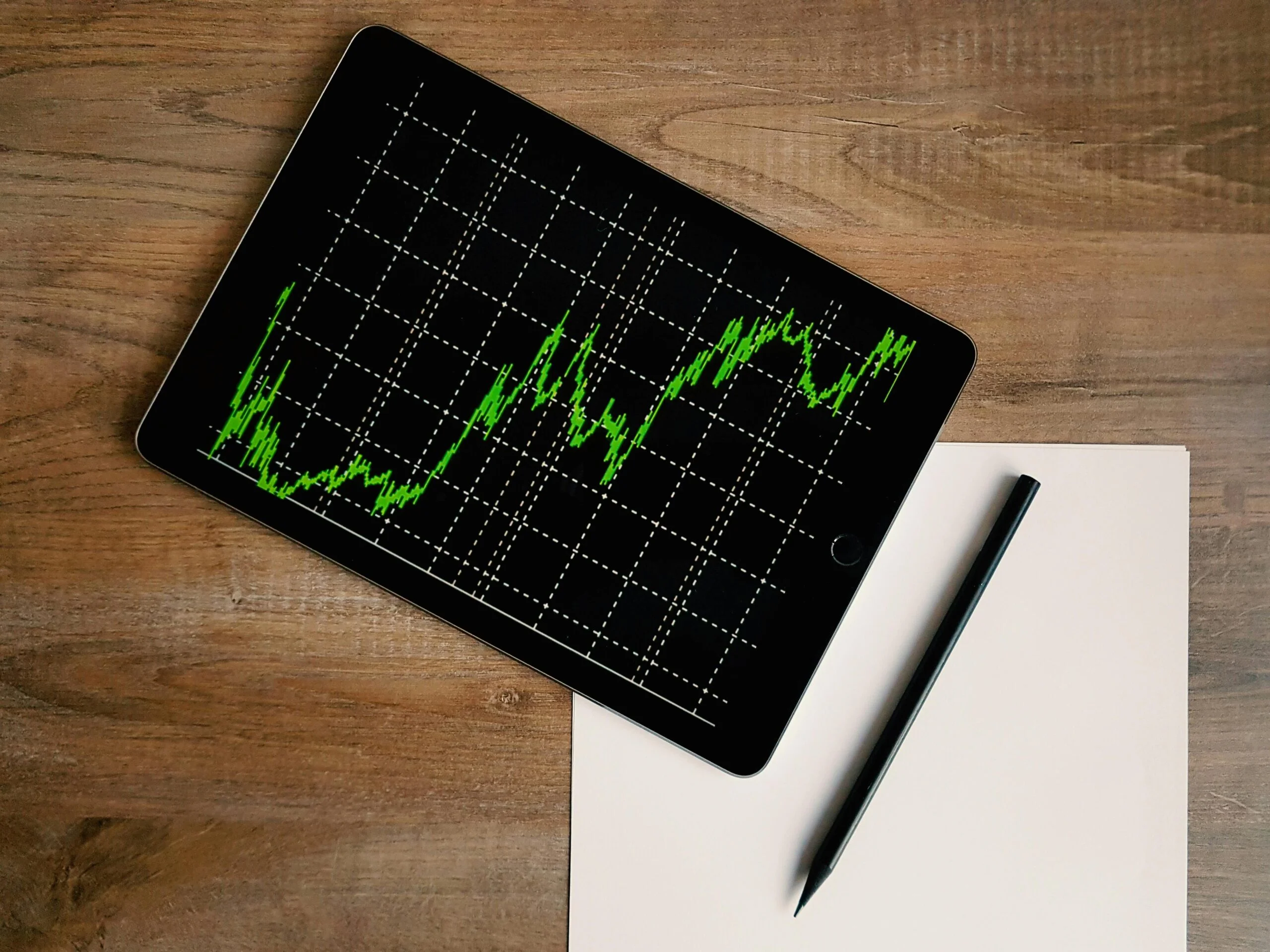
7 Top Data Collection Companies in 2024
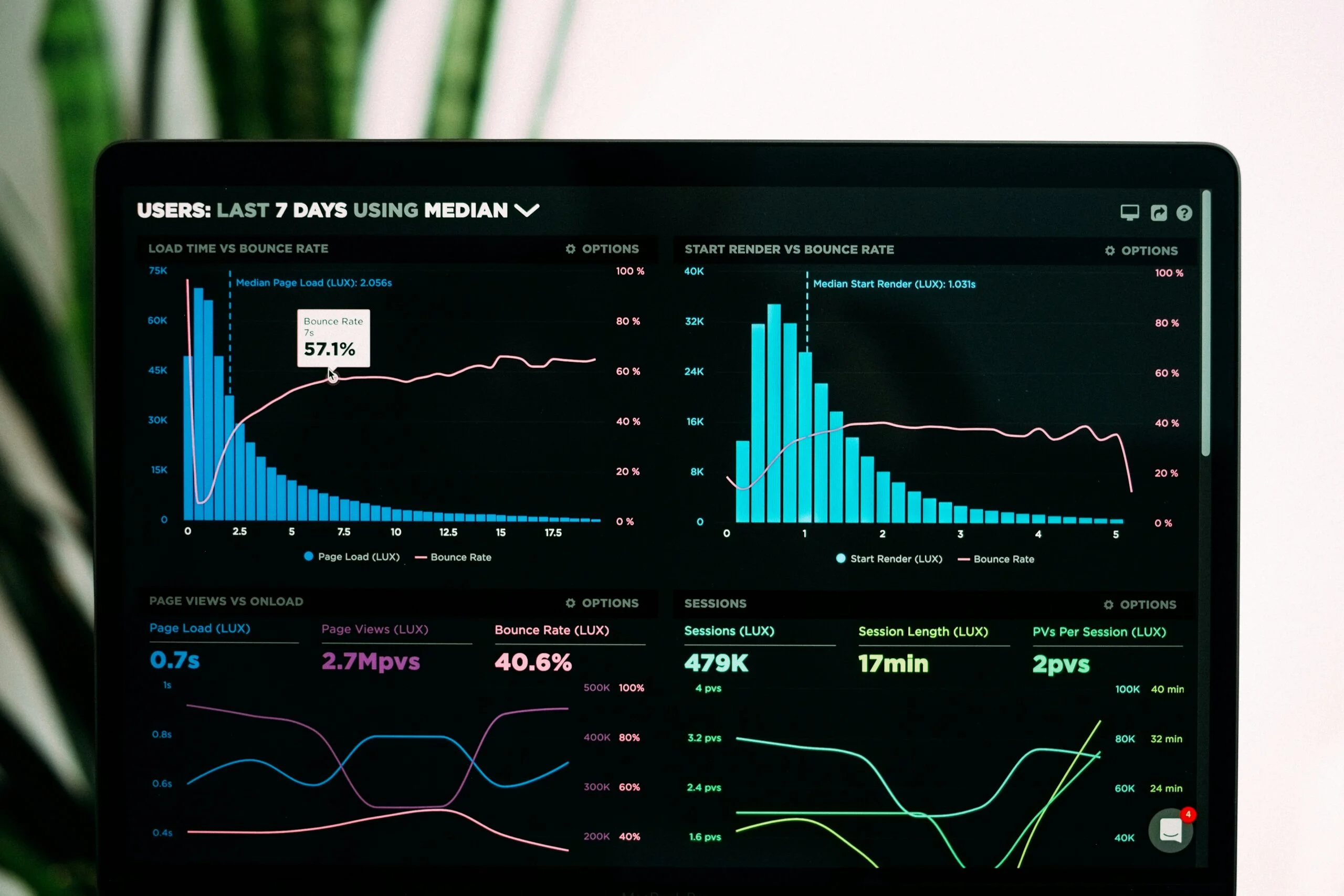
7 Top Data Classification Tools in 2024
Leave a comment cancel reply.
Your email address will not be published. Required fields are marked *
Save my name, email, and website in this browser for the next time I comment.
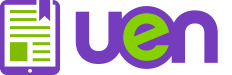
Part II: Data Analysis Methods in Quantitative Research
Data analysis methods in quantitative research.
We started this module with levels of measurement as a way to categorize our data. Data analysis is directed toward answering the original research question and achieving the study purpose (or aim). Now, we are going to delve into two main statistical analyses to describe our data and make inferences about our data:
Descriptive Statistics and Inferential Statistics.
Descriptive Statistics:
Before you panic, we will not be going into statistical analyses very deeply. We want to simply get a good overview of some of the types of general statistical analyses so that it makes some sense to us when we read results in published research articles.
Descriptive statistics summarize or describe the characteristics of a data set. This is a method of simply organizing and describing our data. Why? Because data that are not organized in some fashion are super difficult to interpret.
Let’s say our sample is golden retrievers (population “canines”). Our descriptive statistics tell us more about the same.
- 37% of our sample is male, 43% female
- The mean age is 4 years
- Mode is 6 years
- Median age is 5.5 years
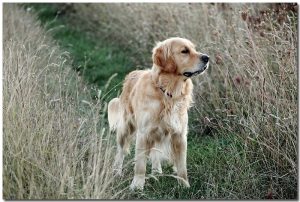
Let’s explore some of the types of descriptive statistics.
Frequency Distributions : A frequency distribution describes the number of observations for each possible value of a measured variable. The numbers are arranged from lowest to highest and features a count of how many times each value occurred.
For example, if 18 students have pet dogs, dog ownership has a frequency of 18.
We might see what other types of pets that students have. Maybe cats, fish, and hamsters. We find that 2 students have hamsters, 9 have fish, 1 has a cat.
You can see that it is very difficult to interpret the various pets into any meaningful interpretation, yes?
Now, let’s take those same pets and place them in a frequency distribution table.
As we can now see, this is much easier to interpret.
Let’s say that we want to know how many books our sample population of students have read in the last year. We collect our data and find this:
We can then take that table and plot it out on a frequency distribution graph. This makes it much easier to see how the numbers are disbursed. Easier on the eyes, yes?
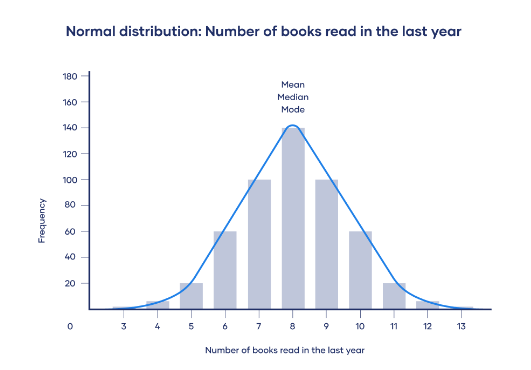
Here’s another example of symmetrical, positive skew, and negative skew:
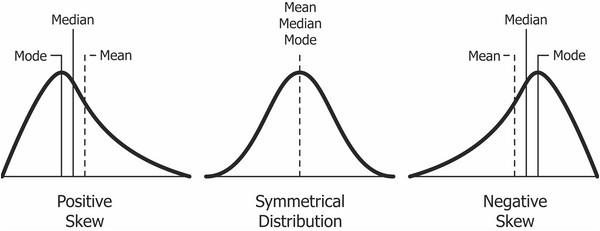
Correlation : Relationships between two research variables are called correlations . Remember, correlation is not cause-and-effect. Correlations simply measure the extent of relationship between two variables. To measure correlation in descriptive statistics, the statistical analysis called Pearson’s correlation coefficient I is often used. You do not need to know how to calculate this for this course. But, do remember that analysis test because you will often see this in published research articles. There really are no set guidelines on what measurement constitutes a “strong” or “weak” correlation, as it really depends on the variables being measured.
However, possible values for correlation coefficients range from -1.00 through .00 to +1.00. A value of +1 means that the two variables are positively correlated, as one variable goes up, the other goes up. A value of r = 0 means that the two variables are not linearly related.
Often, the data will be presented on a scatter plot. Here, we can view the data and there appears to be a straight line (linear) trend between height and weight. The association (or correlation) is positive. That means, that there is a weight increase with height. The Pearson correlation coefficient in this case was r = 0.56.
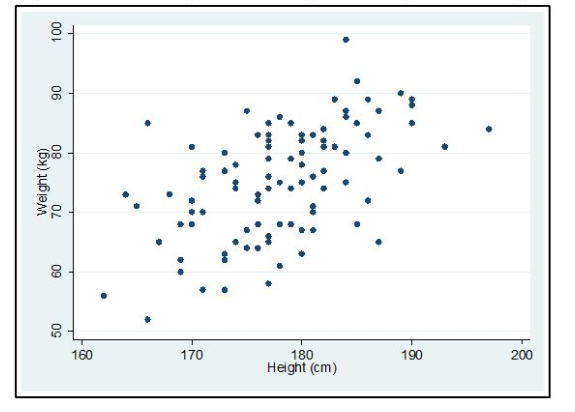
A type I error is made by rejecting a null hypothesis that is true. This means that there was no difference but the researcher concluded that the hypothesis was true.
A type II error is made by accepting that the null hypothesis is true when, in fact, it was false. Meaning there was actually a difference but the researcher did not think their hypothesis was supported.
Hypothesis Testing Procedures : In a general sense, the overall testing of a hypothesis has a systematic methodology. Remember, a hypothesis is an educated guess about the outcome. If we guess wrong, we might set up the tests incorrectly and might get results that are invalid. Sometimes, this is super difficult to get right. The main purpose of statistics is to test a hypothesis.
- Selecting a statistical test. Lots of factors go into this, including levels of measurement of the variables.
- Specifying the level of significance. Usually 0.05 is chosen.
- Computing a test statistic. Lots of software programs to help with this.
- Determining degrees of freedom ( df ). This refers to the number of observations free to vary about a parameter. Computing this is easy (but you don’t need to know how for this course).
- Comparing the test statistic to a theoretical value. Theoretical values exist for all test statistics, which is compared to the study statistics to help establish significance.
Some of the common inferential statistics you will see include:
Comparison tests: Comparison tests look for differences among group means. They can be used to test the effect of a categorical variable on the mean value of some other characteristic.
T-tests are used when comparing the means of precisely two groups (e.g., the average heights of men and women). ANOVA and MANOVA tests are used when comparing the means of more than two groups (e.g., the average heights of children, teenagers, and adults).
- t -tests (compares differences in two groups) – either paired t-test (example: What is the effect of two different test prep programs on the average exam scores for students from the same class?) or independent t-test (example: What is the difference in average exam scores for students from two different schools?)
- analysis of variance (ANOVA, which compares differences in three or more groups) (example: What is the difference in average pain levels among post-surgical patients given three different painkillers?) or MANOVA (compares differences in three or more groups, and 2 or more outcomes) (example: What is the effect of flower species on petal length, petal width, and stem length?)
Correlation tests: Correlation tests check whether variables are related without hypothesizing a cause-and-effect relationship.
- Pearson r (measures the strength and direction of the relationship between two variables) (example: How are latitude and temperature related?)
Nonparametric tests: Non-parametric tests don’t make as many assumptions about the data, and are useful when one or more of the common statistical assumptions are violated. However, the inferences they make aren’t as strong as with parametric tests.
- chi-squared ( X 2 ) test (measures differences in proportions). Chi-square tests are often used to test hypotheses. The chi-square statistic compares the size of any discrepancies between the expected results and the actual results, given the size of the sample and the number of variables in the relationship. For example, the results of tossing a fair coin meet these criteria. We can apply a chi-square test to determine which type of candy is most popular and make sure that our shelves are well stocked. Or maybe you’re a scientist studying the offspring of cats to determine the likelihood of certain genetic traits being passed to a litter of kittens.
Inferential Versus Descriptive Statistics Summary Table
Statistical Significance Versus Clinical Significance
Finally, when it comes to statistical significance in hypothesis testing, the normal probability value in nursing is <0.05. A p=value (probability) is a statistical measurement used to validate a hypothesis against measured data in the study. Meaning, it measures the likelihood that the results were actually observed due to the intervention, or if the results were just due by chance. The p-value, in measuring the probability of obtaining the observed results, assumes the null hypothesis is true.
The lower the p-value, the greater the statistical significance of the observed difference.
In the example earlier about our diabetic patients receiving online diet education, let’s say we had p = 0.05. Would that be a statistically significant result?
If you answered yes, you are correct!
What if our result was p = 0.8?
Not significant. Good job!
That’s pretty straightforward, right? Below 0.05, significant. Over 0.05 not significant.
Could we have significance clinically even if we do not have statistically significant results? Yes. Let’s explore this a bit.
Statistical hypothesis testing provides little information for interpretation purposes. It’s pretty mathematical and we can still get it wrong. Additionally, attaining statistical significance does not really state whether a finding is clinically meaningful. With a large enough sample, even a small very tiny relationship may be statistically significant. But, clinical significance is the practical importance of research. Meaning, we need to ask what the palpable effects may be on the lives of patients or healthcare decisions.
Remember, hypothesis testing cannot prove. It also cannot tell us much other than “yeah, it’s probably likely that there would be some change with this intervention”. Hypothesis testing tells us the likelihood that the outcome was due to an intervention or influence and not just by chance. Also, as nurses and clinicians, we are not concerned with a group of people – we are concerned at the individual, holistic level. The goal of evidence-based practice is to use best evidence for decisions about specific individual needs.
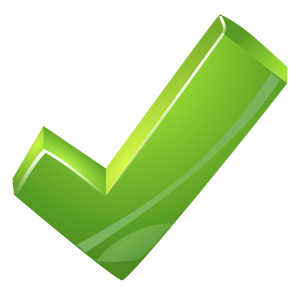
Additionally, begin your Discussion section. What are the implications to practice? Is there little evidence or a lot? Would you recommend additional studies? If so, what type of study would you recommend, and why?
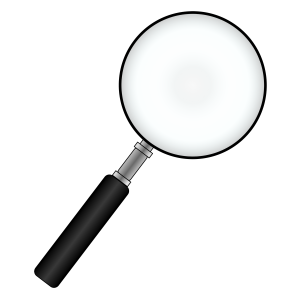
- Were all the important results discussed?
- Did the researchers discuss any study limitations and their possible effects on the credibility of the findings? In discussing limitations, were key threats to the study’s validity and possible biases reviewed? Did the interpretations take limitations into account?
- What types of evidence were offered in support of the interpretation, and was that evidence persuasive? Were results interpreted in light of findings from other studies?
- Did the researchers make any unjustifiable causal inferences? Were alternative explanations for the findings considered? Were the rationales for rejecting these alternatives convincing?
- Did the interpretation consider the precision of the results and/or the magnitude of effects?
- Did the researchers draw any unwarranted conclusions about the generalizability of the results?
- Did the researchers discuss the study’s implications for clinical practice or future nursing research? Did they make specific recommendations?
- If yes, are the stated implications appropriate, given the study’s limitations and the magnitude of the effects as well as evidence from other studies? Are there important implications that the report neglected to include?
- Did the researchers mention or assess clinical significance? Did they make a distinction between statistical and clinical significance?
- If clinical significance was examined, was it assessed in terms of group-level information (e.g., effect sizes) or individual-level results? How was clinical significance operationalized?
References & Attribution
“ Green check mark ” by rawpixel licensed CC0 .
“ Magnifying glass ” by rawpixel licensed CC0
“ Orange flame ” by rawpixel licensed CC0 .
Polit, D. & Beck, C. (2021). Lippincott CoursePoint Enhanced for Polit’s Essentials of Nursing Research (10th ed.). Wolters Kluwer Health
Vaid, N. K. (2019) Statistical performance measures. Medium. https://neeraj-kumar-vaid.medium.com/statistical-performance-measures-12bad66694b7
Evidence-Based Practice & Research Methodologies Copyright © by Tracy Fawns is licensed under a Creative Commons Attribution-NonCommercial-ShareAlike 4.0 International License , except where otherwise noted.
Share This Book
- University Libraries
- Research Guides
- Topic Guides
- Research Methods Guide
- Data Analysis
Research Methods Guide: Data Analysis
- Introduction
- Research Design & Method
- Survey Research
- Interview Research
- Resources & Consultation
Tools for Analyzing Survey Data
- R (open source)
- Stata
- DataCracker (free up to 100 responses per survey)
- SurveyMonkey (free up to 100 responses per survey)
Tools for Analyzing Interview Data
- AQUAD (open source)
- NVivo
Data Analysis and Presentation Techniques that Apply to both Survey and Interview Research
- Create a documentation of the data and the process of data collection.
- Analyze the data rather than just describing it - use it to tell a story that focuses on answering the research question.
- Use charts or tables to help the reader understand the data and then highlight the most interesting findings.
- Don’t get bogged down in the detail - tell the reader about the main themes as they relate to the research question, rather than reporting everything that survey respondents or interviewees said.
- State that ‘most people said …’ or ‘few people felt …’ rather than giving the number of people who said a particular thing.
- Use brief quotes where these illustrate a particular point really well.
- Respect confidentiality - you could attribute a quote to 'a faculty member', ‘a student’, or 'a customer' rather than ‘Dr. Nicholls.'
Survey Data Analysis
- If you used an online survey, the software will automatically collate the data – you will just need to download the data, for example as a spreadsheet.
- If you used a paper questionnaire, you will need to manually transfer the responses from the questionnaires into a spreadsheet. Put each question number as a column heading, and use one row for each person’s answers. Then assign each possible answer a number or ‘code’.
- When all the data is present and correct, calculate how many people selected each response.
- Once you have calculated how many people selected each response, you can set up tables and/or graph to display the data. This could take the form of a table or chart.
- In addition to descriptive statistics that characterize findings from your survey, you can use statistical and analytical reporting techniques if needed.
Interview Data Analysis
- Data Reduction and Organization: Try not to feel overwhelmed by quantity of information that has been collected from interviews- a one-hour interview can generate 20 to 25 pages of single-spaced text. Once you start organizing your fieldwork notes around themes, you can easily identify which part of your data to be used for further analysis.
- What were the main issues or themes that struck you in this contact / interviewee?"
- Was there anything else that struck you as salient, interesting, illuminating or important in this contact / interviewee?
- What information did you get (or failed to get) on each of the target questions you had for this contact / interviewee?
- Connection of the data: You can connect data around themes and concepts - then you can show how one concept may influence another.
- Examination of Relationships: Examining relationships is the centerpiece of the analytic process, because it allows you to move from simple description of the people and settings to explanations of why things happened as they did with those people in that setting.
- << Previous: Interview Research
- Next: Resources & Consultation >>
- Last Updated: Aug 21, 2023 10:42 AM

IMAGES
VIDEO
COMMENTS
Data analysis is a crucial step in the research process, transforming raw data into meaningful insights that drive informed decisions and advance knowledge. This article explores the various types and methods of data analysis in research, providing a comprehensive guide for researchers across disciplines. Data Analysis in Research
Learn how to analyze data in research using different methods and techniques for qualitative and quantitative data. Find out the definition, purpose, types, and examples of data analysis in research.
Learn the basics of data analysis, from quantitative to qualitative, from mathematical to artificial intelligence. Explore the tree diagram of data analysis types, methods, and techniques with examples and definitions.
By understanding the process, methods, and types of data analysis, researchers and professionals can effectively tackle complex problems, uncover trends, and make data-driven decisions. With advancements in tools and technology, the scope and impact of data analysis continue to expand, shaping the future of industries and research.
Learn what data analysis is, why it is important, and how to use different types of data analysis techniques. Explore the distinction between quantitative and qualitative data, and the tools for data analysis.
Another important thing that is still related to research methods and data collection techniques is data analysis techniques. The data analysis used determines the meaningfulness of the collected ...
In scientific research, statistical methods ensure accurate results by validating hypotheses and analyzing experimental data. Methods such as regression analysis and ANOVA (analysis of variance) allow researchers to draw conclusions from experiments by examining relationships between variables and identifying key factors that influence outcomes.
Whether it's improving business operations or advancing academic research, data analysis methods and techniques are the cornerstone of informed action. Importance of Data Analysis Methods and Techniques. Implementing the correct data analysis methods is vital for: Accurate Insights: Ensuring data is interpreted correctly to make sound decisions.
Data Analysis Methods in Quantitative Research. We started this module with levels of measurement as a way to categorize our data. Data analysis is directed toward answering the original research question and achieving the study purpose (or aim). Now, we are going to delve into two main statistical analyses to describe our data and make ...
Data Analysis and Presentation Techniques that Apply to both Survey and Interview Research Create a documentation of the data and the process of data collection. Analyze the data rather than just describing it - use it to tell a story that focuses on answering the research question.